Digital Twins and Simulation: A New Era in Modeling
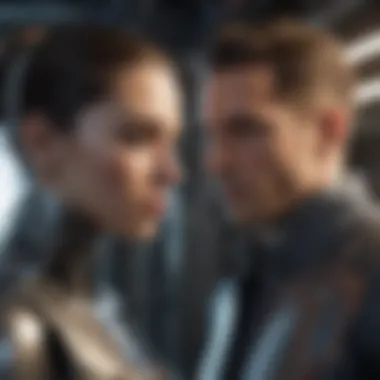
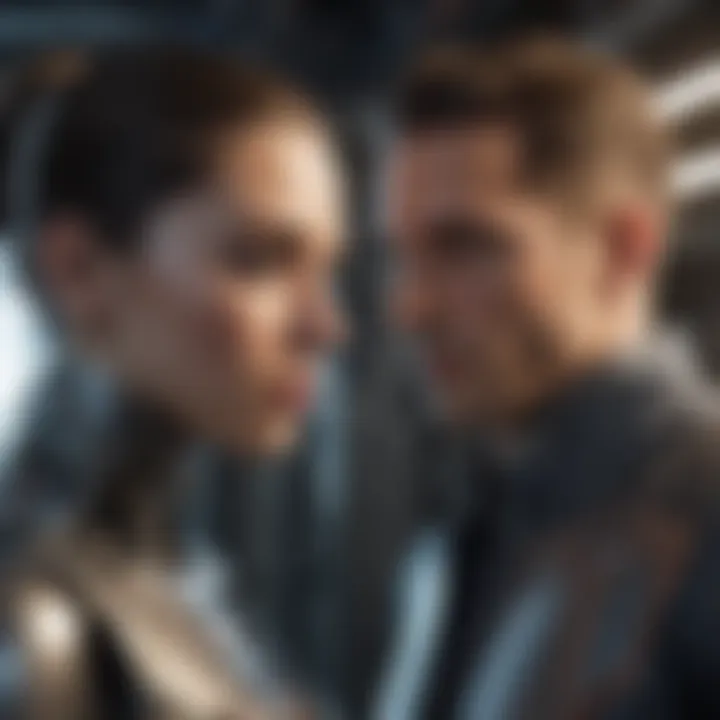
Intro
The fusion of the digital realm with physical realities has given rise to concepts that redefine how we understand and engage with our environments. Among these concepts, digital twins and simulation emerge as fundamental pillars, enhancing industries ranging from manufacturing to healthcare. This intricate interplay, marked by a robust synergy, allows for advanced modeling techniques that not only improve decision-making but also innovate processes and enhance operational efficiencies.
Understanding these tools starts with grasping their definitions and implications. Digital twins represent virtual replicas of physical entities or systems, leveraging real-time data to mirror their state, behaviors, and dynamics. Conversely, simulation refers to the process of creating a model to imitate the operation of real-world processes or systems over time. Together, they create a landscape rich with potential, driving industries towards a future where optimization and efficiency reign supreme.
By peeling back the layers of these concepts, we uncover a narrative filled with progress and innovation, underscoring their critical role in modern technological landscapes.
Prelims to Digital Twins
In an age where technology is reshaping how we approach design, manufacturing, and operational processes, the concept of digital twins emerges as a pivotal element. This topic is not just a fad; it stands at the intersection of data analytics, IoT (Internet of Things), and simulation technologies. Digital twins allow us to create real-time digital replicas of physical entities, paving the way for better decision-making and efficiency in multiple sectors.
The importance of understanding digital twins lies in their ability to provide insights that traditional modeling and simulation cannot achieve on their own. At their core, digital twins act as a bridge between the physical and the virtual, enabling users to monitor, analyze, and optimize systems proactively. For industries grappling with complex processes and rapid technological advancements, adopting digital twin frameworks is not merely beneficial; it’s essential.
The factors that make exploring the concept of digital twins vital include:
- Performance Enhancement : Digital twins improve performance through predictive maintenance, minimizing downtime and maximizing productivity.
- Resource Efficiency: They enable better resource management by simulating different scenarios and analyzing outcomes without impacting real-world operations.
- Innovation Catalyst: Organizations leverage these tools to innovate set processes or develop new applications, pushing boundaries that were previously thought to be rigid.
- Risk Mitigation: By simulating diverse situations, businesses can identify potential risks and develop strategies to tackle them effectively.
As we delve deeper, it becomes vital to clarify what precisely constitutes a digital twin. Knowing its definition will pave the way for understanding its historical context, features, and relevance in various applications.
Core Elements of the Digital Twin Framework
The framework of digital twins is pivotal for understanding their practical implications across various sectors. This framework delineates the characteristic components that contribute to the effectiveness and functionality of a digital twin, acting essentially as the backbone of innovative modeling. At its core, it involves three crucial elements: data requirements and integration, modeling techniques, and the presence of feedback loops. Each of these components holds significant weight in ensuring that digital twins can provide accurate simulations and insights, thereby enhancing decision-making processes.
Data Requirements and Integration
Data forms the lifeblood of digital twins. The essence of utilizing a digital twin hinges on how effectively data can be gathered, processed, and integrated from real-world systems. The data requirements encompass not only the volume but also the quality of information used to create a reliable twin. This can include historical performance data, real-time sensor data, or even operational metrics from various sources.
A key challenge in this integration is ensuring interoperability across diverse systems. Different devices might generate data in assorted formats and protocols. Consequently, establishing a seamless flow of information is paramount. This can be achieved through robust API strategies and standardized data formats, enabling real-time communication between physical and digital counterparts.
Integrating advanced analytics and machine learning algorithms also plays a significant role in enhancing data utility. By extracting meaningful insights from raw data, organizations can transform mere numbers into actionable intelligence.
Modeling Techniques
When it comes to modeling, there’s no one-size-fits-all solution. Various techniques apply depending on the nature of the system being modeled and the specific objectives an organization aims to achieve. Some of the widely adopted modeling techniques include physics-based models, data-driven models, and hybrid approaches that leverage both methodologies.
1. Physics-Based Models: These models rely on the fundamental laws of physics to simulate real-world behavior. They are particularly useful in scenarios where understanding physical forces or interactions is crucial, such as in aerospace or automotive engineering.
2. Data-Driven Models: In contrast, data-driven models depend solely on the statistical relationships found in historical data. They are valuable in contexts where physical laws are challenging to ascertain or less relevant, such as in financial predictions or marketing analytics.
3. Hybrid Models: Combining elements from both physics-based and data-driven techniques can yield significant advantages. Such hybrid models can offer a more nuanced and accurate representation of complex systems, making them particularly beneficial in situations where data is sparse or noise-laden.
Feedback Loops in Digital Twins
One of the distinguishing features of digital twins is their ability to utilize feedback loops to enhance performance. A feedback loop allows the twin to receive information from its physical counterpart, facilitating continuous learning and adaptation. This dynamic interaction not only improves the accuracy of the simulations but also informs decision-making in real-time.
For instance, consider a manufacturing plant equipped with multiple digital twins representing different machinery. Changes in operational parameters, like temperature or pressure, can be monitored. These parameters are fed back to the digital twin, which adjusts its model accordingly. As a result, it provides updated recommendations on maintenance schedules or operational shifts based on current conditions.
This feedback mechanism is beneficial in various applications, including predictive maintenance, where anticipating equipment failures can save resources and reduce downtimes. By using feedback loops effectively, organizations can also enhance their innovation processes, allowing for rapid iterations and adjustments based on immediate insights.
"In an era where data generation is ubiquitous, effective integration and utilization within the digital twin framework can unlock vast potential for industries."
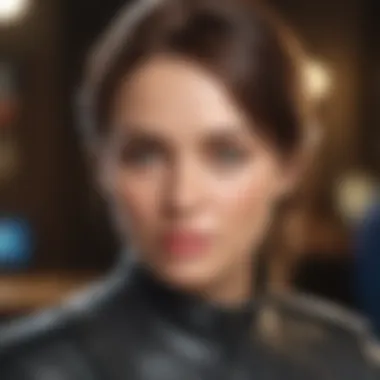
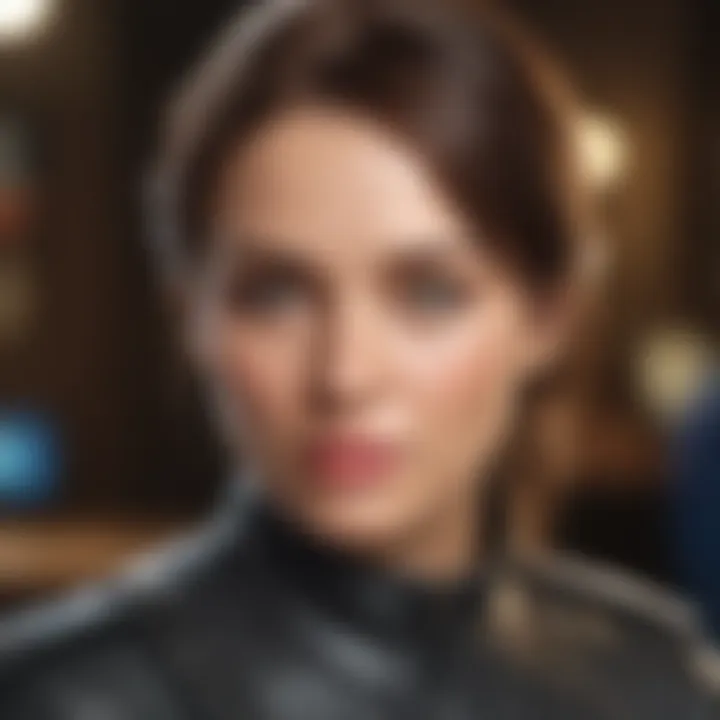
Through exploring these core elements of the digital twin framework, we gain a fundamental understanding of how they collectively facilitate advanced modeling, enabling deeper insights and more informed decision-making.
Understanding Simulation in Context
Understanding the role of simulation in today's ever-evolving tech landscape is paramount. It forms the backbone of numerous breakthroughs in diverse sectors, acting as a virtual testing ground where theories and hypotheses undergo rigorous scrutiny. With the advent of digital twins, the relevance of simulation has grown even stronger, providing enriched layers of analysis and prediction.
The main appeal of simulation lies in its ability to mimic real-world processes without the inherent risks or costs associated with physical experimentation. For instance, in aircraft engineering, simulations can calculate various design efficiencies or safety parameters before the first prototype ever flies. This risk management is particularly crucial in industries where human safety is on the line or where the cost of failure can be detrimental.
Types of Simulations
Simulations can be classified in various ways, depending on their purpose, methodology, and application. Here are some primary types:
- Discrete Event Simulation (DES): This type of simulation represents systems as a sequence of events where each event occurs at a specific point in time. For example, in logistics, DES can help optimize inventory levels by simulating how products move through a warehouse.
- Continuous Simulation: These simulations study systems that change continuously over time. They often apply in environmental modeling, assessing temperature changes over a period, or data flow in network systems.
- Monte Carlo Simulation: Monte Carlo simulations leverage random sampling to calculate probabilities and forecast outcomes. It’s widely used in finance for risk assessment related to investments.
- System Dynamics: System dynamics simulations focus on the behavior of complex systems over time, often using differential equations to represent process interactions. This can be invaluable in assessing long-term environmental policies’ effectiveness.
- Agent-Based Modeling: This type explores how individual components (agents) within a system interact with each other and their environment, often leading to emergent behaviors at the macro scale. For instance, traffic flow analysis utilizes agent-based modeling to illustrate how individual vehicles behave in response to changing conditions.
Each of these types has distinct strengths, making them suited to different scenarios, and their design often hinges on both the desired outcomes and initial conditions of the real-world processes they aim to replicate.
Comparative Analysis: Simulation vs. Reality
To truly grasp the capabilities of simulation, it's important to compare it to reality itself. While nothing truly can replace the tangible unpredictability and nuances of real-life scenarios, simulations offer valuable insights that can often lead to enhanced understanding and improved decision-making.
- Cost-effectiveness: Running a simulation can be far less expensive than undertaking large-scale tests. For example, drug testing in pharmaceuticals often begins with simulations before any real-life testing, which not only saves money but also minimizes researcher exposure to potentially harmful substances.
- Time efficiency: Simulations allow stakeholders to run multiple scenarios in a fraction of the time required for real-world experiments. An automotive manufacturer can test dozens of crash scenarios via simulation in just a few hours, while actual crash tests take substantially longer to coordinate.
- Accuracy in prediction: Advanced simulations can offer highly precise modeling based on extensive datasets. However, real-world variables, like unforeseen human behavior or environmental factors, can deviate the results from predicted outcomes. In other words, while simulations can guide expectations, they might not always perfectly predict the future.
- Understanding complexity: Complex scenarios, such as climate modeling or market behavior, can overwhelm human intuition. However, simulation provides structured frameworks that dissect complexity into understandable components, revealing interdependencies.
In summary, while simulations may not perfectly encapsulate reality, they prove invaluable for forecasting, planning, and optimizing processes across various fields. Their integration with digital twins amplifies their effectiveness, paving the way for more informed decision-making and innovative advancements.
"Simulation is the bridge between the theoretical and the practical, offering a platform to explore possibilities that reality often constrains."
Through this interplay, industries stand to gain immeasurably, adopting more agile and strategic approaches to challenges, thus shaping the future landscape of their operations.
Interrelationship Between Digital Twins and Simulation
The relationship between digital twins and simulation is akin to a two-way street, where advancements in one area profoundly impact the other. As businesses increasingly turn to digital twins, they leverage the power of simulation to experiment with potential outcomes before any real-world implementation. This synergy not only enhances the accuracy of simulations but also opens up new avenues for innovation in various industries.
How Digital Twins Enhance Simulations
Digital twins act as mirrors reflecting the real-time status of their physical counterparts. This feature provides simulations with data that is not just theoretical but grounded in reality. When simulating processes or systems, such as production lines in manufacturing or patient flows in healthcare, having access to accurate, up-to-the-minute information ensures that simulations yield applicable results.
- Real-Time Data: Digital twins continuously gather and feed real-time data to simulations. This data includes environmental factors, user interactions, and operational states.
- Dynamic Modeling: Changes in conditions can be quickly reflected in the simulations. This adaptability allows industries to refine their strategies efficiently, reducing trial-and-error time.
- Predictive Insights: With simulations powered by digital twin data, organizations can foresee potential challenges and devise plans before issues actually arise.
This capability holds immense significance, especially for sectors where errors could be disastrous. For instance, in aerospace, simulating flight paths with real-time environmental data from digital twins can enhance safety measures incredibly.
Validating Simulations with Digital Twins
Conversely, digital twins also play a pivotal role in validating simulation outcomes. When a simulation proposes a solution to a problem, how do we know it’s accurate? That’s where digital twins step in, serving as verification models.
- Comparative Analysis: By comparing the results from simulations with actual performance data from digital twins, organizations can ascertain the reliability of predicted outcomes.
- Feedback Mechanism: The correlation works both ways; validation allows for improvements to be made in both the digital twin and the simulation models, creating a cycle of enhancement.
- Risk Management: Digital twins provide a way to test scenarios without the inherent risks that come with physical trials. In areas like urban planning, predicting how traffic might flow can lead to adjustments before building any new infrastructure.
"The integration of digital twins and simulations is not just about surviving in a competitive environment but thriving in it."
In summary, digital twins and simulations feed off each other, creating a feedback loop that enhances capabilities while minimizing potential risks. They allow industries to pivot quickly, making informed decisions based on validated outcomes, ultimately pushing boundaries in innovation and efficiency.
Applications Across Various Industries
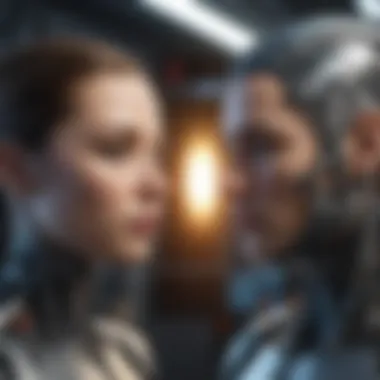
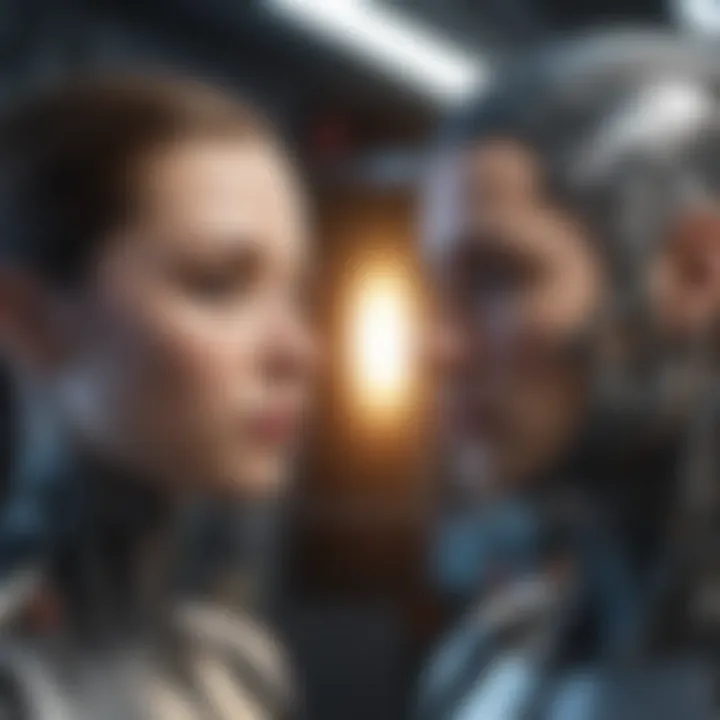
In the landscape of Industry 4.0, digital twins and simulation are becoming indispensable tools across various sectors. Their integration brings unique advantages by enabling a more nuanced understanding of operations, facilitating predictive maintenance, and fostering innovation. By mapping real-world entities into the digital domain, industries can optimize processes and improve decision-making. Let’s explore how this synergy plays out in critical sectors such as manufacturing, healthcare, urban development, and aerospace.
Manufacturing and Supply Chain
The manufacturing sector has made significant strides with the application of digital twins. These virtual replicas allow companies to monitor equipment performance in real-time, identify potential faults before they lead to failures, and optimize production lines. For instance, using digital twins, organizations such as Siemens have been able to simulate entire factories to detect bottlenecks and enhance throughput efficiency.
Moreover, digital twins play a vital role in the supply chain by providing a comprehensive view of logistics. Businesses can track products from point A to point B, visualize inventory levels, and even forecast demand more accurately. By employing simulations, organizations can test various scenarios, such as disruptions or changes in demand, and plan accordingly.
Healthcare Innovations
In healthcare, the integration of digital twins is revolutionizing patient care. These models can represent the unique characteristics of a patient, including their medical history and genetic data, allowing for personalized treatment plans. For example, hospitals are starting to use digital twins to simulate surgeries before actual procedures. This not only enhances the surgeon's confidence but can also lead to better patient outcomes.
The pandemic has bolstered the use of simulation in healthcare. By modeling disease spread and responses to interventions, healthcare professionals can devise and optimize strategies to contain diseases. This capability is proving essential in maintaining public health while minimizing impacts on healthcare systems.
Urban Development and Smart Cities
As cities grow and evolve, planners must tackle increasing demands for infrastructure, utilities, and public services. Digital twins offer city planners a powerful tool for creating smart cities. For example, by simulating traffic patterns, urban developers can design better transit systems that reduce congestion and pollution.
Moreover, integrating municipal data into these digital models allows for real-time monitoring of urban resources, from water usage to waste management, thus promoting sustainability. Data-driven insights enable smart management of utility services, ultimately improving the quality of life for residents.
Aerospace and Defense Applications
The aerospace and defense sectors are at the forefront of adopting digital twins. Companies such as Boeing are using these technologies to model aircraft systems, monitor performance throughout the lifecycle, and predict maintenance needs. This proactive approach not only reduces costs but also enhances safety and reliability.
Simulation is equally crucial in defense, where scenarios can be modeled to train personnel and strategize for various operational conditions. Digital twins facilitate virtual exercises that mirror real-world challenges, enabling better preparedness without the associated risks of live drills.
The integration of digital twins across industries demonstrates a shift towards more data-driven decision-making processes, pushing the boundaries of what’s possible in operational efficiency and innovation.
The applications of digital twins and simulations cut across various industries, each harnessing these technologies to improve performance, enhance safety, and drive creativity. As these fields develop, the potential for innovation continues to expand, making the future of digital twins and simulation a promising landscape.
Challenges and Considerations
Understanding the challenges and considerations surrounding digital twins and simulation is crucial to maximizing their potential. These issues can either serve as a hindrance or pave the way for more sophisticated applications in industries today. Addressing these challenges ensures that the integration of these technologies is not just a fleeting trend but a foundational element that can enhance long-term operational efficiency and innovation.
Data Privacy and Security Issues
The advent of digital twins leads to a treasure trove of data, but this also opens a can of worms regarding privacy and security. Each digital twin represents a real-world counterpart, collecting vast amounts of sensitive information.
Data breaches have become increasingly common, and organizations must prioritize safeguarding this information. Key steps to ensure data security include:
- Adopting stringent encryption techniques: Protecting data at rest and in transit is vital.
- Establishing clear access controls: Not everyone needs the same level of access to sensitive data.
- Conducting regular security audits: Ensuring that the systems are regularly tested against vulnerabilities.
Moreover, companies must comply with regulations like GDPR, which mandates transparent practices around personal data usage. Failure to address these privacy issues can result in hefty fines and loss of trust. Ultimately, prioritizing data privacy not only protects the organization but also enhances stakeholder confidence.
"A digital twin is only as trustworthy as the integrity of its data."
Interoperability Among Different Systems
Another challenge that pops up is alignment among varied systems. In the world of digital twins, interoperability is key for seamless communication between platforms. Different systems often use unique protocols, which can hinder the overall efficacy of the digital twin approach.
For organizations to truly exploit these technologies, they need:
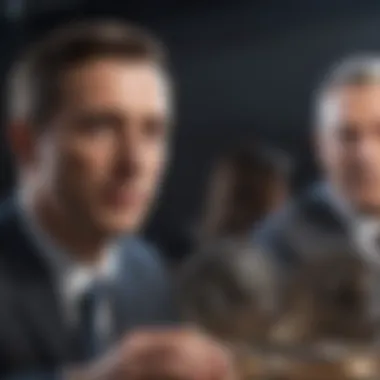
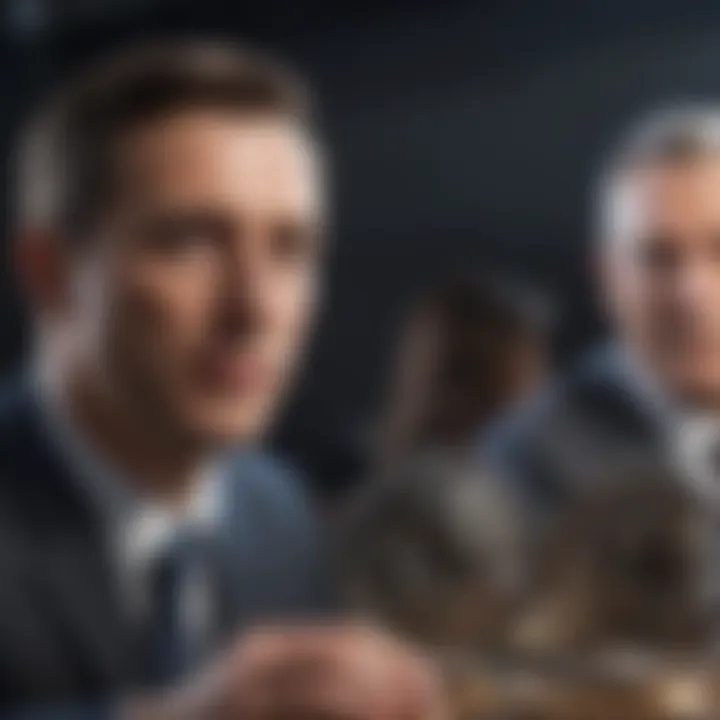
- Standardized protocols: The industry should rally behind common standards to allow systems to communicate effectively.
- Robust API frameworks: These can facilitate smoother data exchange between diverse systems.
- Collaborative efforts: Teams across the organization must work hand-in-hand to ensure compatibility and make integrations more manageable.
Inadequate interoperability leads to wasted resources and can frustrate users, who may find themselves battling with disjointed systems. Thus, navigating this obstacle is imperative for unlocking the full power of digital twins and simulation.
Scalability and Cost Factors
Scaling a digital twin system isn’t always smooth sailing. As an organization grows, its needs evolve, and the technology must keep pace. This raises questions about the cost implications of scaling.
Key considerations for scalability include:
- Infrastructure Costs: Investing in sufficient computing power and storage capabilities.
- Licensing Fees: Depending on the platform, costs can balloon as more users access the system.
- Training and Support: Employees often need training to adapt to new technologies, which adds another layer of expense.
Understanding these factors is crucial for long-term success. If not addressed early on, scaling issues can lead to performance bottlenecks and limit the organization’s ability to innovate. The financial implications of poorly planned scalability can be significant, impacting company resources and stifling growth.
By keeping a keen eye on these challenges and embracing strategic solutions, companies can more effectively harness the potential of digital twins and simulation technologies.
Future of Digital Twin Technology and Simulation
In considering the Future of Digital Twin Technology and Simulation, we step into a realm of possibilities that promises to redefine how industries operate. This section emphasizes the importance of understanding emerging trends and forecasting their implications, which is essential for organizations looking to stay ahead in an increasingly competitive landscape. The integration of digital twins and simulations isn’t just about enhancing existing frameworks; it's about fundamentally reshaping how we approach operational modeling, decision making, and strategic planning.
Emerging Trends and Forecasts
As we move deeper into the 21st century, we notice several trends breathing new life into the digital twin ecosystem:
- AI and Machine Learning Integration: Organizations are embracing AI to analyze vast datasets captured from physical assets. Machine learning algorithms are being employed to enhance predictive models, allowing digital twins to provide deeper insights and forecasts that were previously unattainable.
- Enhanced Connectivity with IoT: The Internet of Things (IoT) is a game-changer. With devices connected in real-time, digital twins can reflect the current state of operations more accurately, leading to improved response times and better resource allocation.
- Cloud Computing Advantages: Utilizing cloud infrastructure offers scalability and accessibility. More companies are leaning towards cloud-based solutions enabling seamless integration of simulations and real-time data, which creates efficient workflows.
- Focus on Sustainability: As global concerns regarding environmental impact heighten, digital twins are being used to optimize resource use. By simulating various scenarios, organizations can identify the most sustainable practices.
"The convergence of digital twins and advanced simulations is not merely a trend; rather, it's set to drive innovation and operational excellence in the coming decade."
Such advancements are not just a collection of buzzwords; they promise real benefits. For example, industries like automotive and aerospace are already integrating these elements, leading to faster design cycles and more efficient manufacturing processes.
Long-term Impact on Industries
The long-term impacts of digital twin technology and simulation on various industries are profound:
- Improved Operational Efficiency: The precision offered by digital twins means that any inefficiencies can be identified and addressed swiftly. Companies can run simulations to optimize processes before making changes in the physical world, thus saving time and resources.
- Fostering Innovation: Continuous ability to simulate different scenarios encourages creativity. For instance, in product development, engineers can model various design iterations without the costly implications of physical prototypes.
- Risk Management: Predictive capabilities enable organizations to anticipate breakdowns or failures, enhancing safety protocols and reducing downtime. Industries such as oil and gas and manufacturing stand to gain significantly in this aspect.
- Data-Driven Decisions: With reliable simulations backed by real data, strategic decisions can shift from guesswork to informed actions. This leads to more consistent outcomes and ultimately drives success.
- Customization and Personalization: In sectors like healthcare, digital twins can lead to tailored approaches to patient treatment, enhancing patient care based on individual health data.
As we look to the future, it’s clear that the fusion of digital twins and simulation technologies will forge new pathways in various sectors, enabling them to meet challenges with intelligence and agility. Organizations that recognize and leverage these technologies will not only thrive but will also lead the charge toward innovation in their respective fields.
End
The integration of digital twins and simulation is more than just a technological trend; it's a transformational shift impacting various industries and shaping our understanding of complex systems. As this article has explored, the synergy created by linking these two concepts is critical for advanced modeling, offering numerous benefits that extend well beyond traditional boundaries.
Summary of Findings
In summarizing the key insights:
- Digital Twins allow real-time data mirroring of physical entities,
- Simulations provide predictive analysis and scenario testing,
- Together, they enhance decision-making processes, enabling proactive management and optimization.
Both tools promote efficiencies, reduce costs, and drive innovations in sectors like manufacturing, healthcare, urban development, and aerospace. The extensive applications highlight their versatility and potential to revolutionize operational frameworks.
Moreover, addressing challenges regarding data security, interoperability, and scalability is essential for ensuring successful implementation across these domains. This pro-active engagement fosters an environment where technology can flourish, breeding innovative solutions that continually push the envelope of what’s possible.
Final Thoughts
Looking ahead, the future of digital twin technology and simulation appears contiguous with advancements in artificial intelligence, machine learning, and big data analytics. The emphasis on collaborative ecosystems designed to enhance these technologies will likely yield further breakthroughs.
As industries embrace this integration, it becomes imperative for stakeholders to remain vigilant about ethical practices and data governance. The journey towards more interconnected, data-driven environments will take time, but the outcomes promise to redefine operational paradigms.
To that end, the development of training resources and community engagement will be vital as more professionals navigate these technologies across various sectors. Digital twins and simulations stand to both empower and challenge industries in this rapidly evolving landscape.
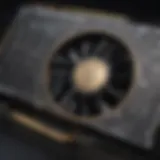
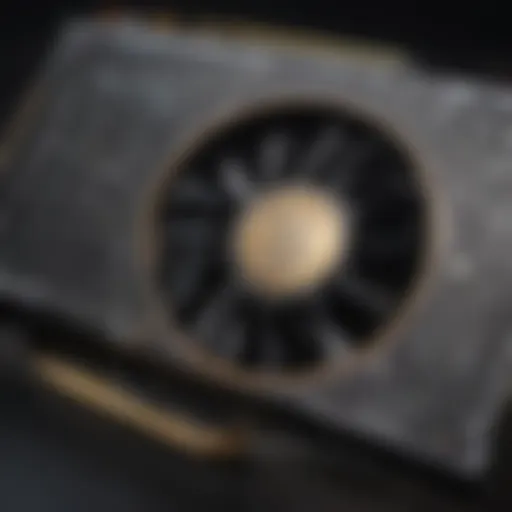