Unraveling the Complexities of Deciphering Random Chart Patterns
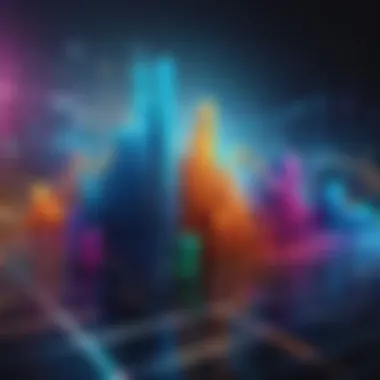
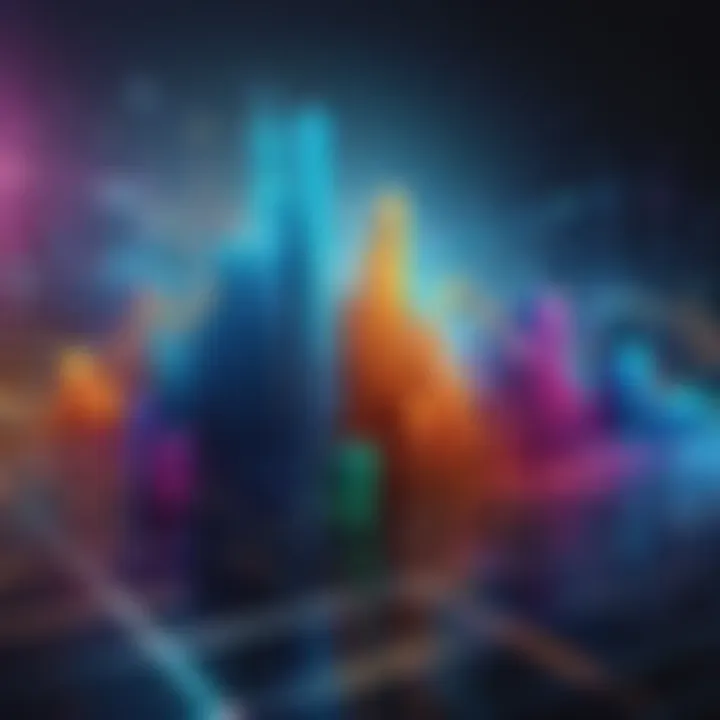
What is random chart:
Random charts are graphical representations of data points or values that are generated through a random process. They are commonly used in various fields such as statistics, finance, and analytics to visualize and analyze random variations. The concept of random charts dates back to the early development of statistical analysis, with notable contributions from pioneers such as Sir Francis Galton and Karl Pearson.
In the realm of cryptocurrencies, random charts play a crucial role in depicting price movements and trends of various digital assets. Traders and investors rely on these charts to make informed decisions regarding buying and selling cryptocurrencies. The randomness in these charts reflects the inherent volatility and unpredictability of the crypto market.
There are numerous types of random charts used in the crypto space, including line charts, candlestick charts, and bar charts, each offering unique insights into price movements. These charts serve the purpose of providing a visual representation of historical price data, allowing users to identify patterns, trends, and potential trading opportunities.
The tokenomics of random charts depend on key tokens such as Bitcoin, Ethereum, and other major cryptocurrencies. These tokens influence the value and volatility of random charts, shaping the trading environment and investment decisions within the crypto ecosystem.
In addition to tokens, the random chart ecosystem incorporates a range of analytical tools and indicators, including moving averages, RSI (Relative Strength Index), and MACD (Moving Average Convergence Divergence). These tools help traders and analysts interpret random charts more effectively, providing valuable insights into market trends and potential price movements.
Introduction
Random chart analysis is a crucial aspect of deciphering complex data patterns and extracting valuable insights. In this article, we aim to delve deep into the intricacies of random chart analysis, shedding light on methodologies and techniques that offer a deeper understanding of this specialized field. By exploring the nuances of random charts, we can uncover hidden patterns and trends that hold significance across various industries and statistical studies.
Defining Random Charts
When it comes to defining random charts, we are looking at a key component in the realm of data analysis. The characteristics of random charts play a vital role in identifying and interpreting data patterns effectively. Understanding these characteristics allows analysts to navigate through vast amounts of random data with precision and accuracy. By examining the purpose of analyzing random data, we can pinpoint specific goals and objectives in conducting in-depth investigations of data sets. The purpose serves as a guiding force in uncovering meaningful insights that drive decision-making processes across different domains.
Characteristics of Random Charts
Random charts exhibit a unique attribute in their data distribution, often displaying unpredictable patterns that challenge analysts to discern underlying trends. This unpredictability presents both challenges and opportunities in exploring new data landscapes. One key characteristic of random charts is their ability to showcase the inherent randomness that exists within datasets. By embracing this randomness, analysts can adopt innovative approaches to pattern recognition and statistical modeling. While the lack of apparent structure may seem daunting, it opens doors to novel analytical methodologies that thrive on uncertainty.
Purpose of Analyzing Random Data
The purpose of analyzing random data lies in uncovering hidden insights that traditional data analysis methods might overlook. By delving into seemingly chaotic datasets, analysts can extract valuable information that could revolutionize decision-making processes. The unique feature of analyzing random data is the potential to discover unexpected correlations and dependencies that lead to groundbreaking discoveries. While the process may pose challenges in terms of data cleaning and normalization, the advantages of unearthing novel insights far outweigh the initial complexities.
Significance of Random Chart Analysis
The significance of random chart analysis extends beyond its application in various industries to its pivotal role in advancing statistical studies. Exploring the applications of random chart analysis across diverse sectors offers a glimpse into the transformative power of data analytics. Similarly, understanding its role in statistical studies sheds light on how random charts contribute to broader research initiatives and scientific endeavors.
Applications in Various Industries
Random chart analysis finds widespread applications in industries spanning finance, healthcare, and marketing, among others. Its adaptability to diverse datasets and its capacity to uncover hidden trends make it a valuable tool for decision-makers seeking data-driven solutions. The key characteristic of random chart analysis in industries is its ability to provide actionable insights that drive innovation and competitive advantage. Leveraging this analytical approach enables businesses to optimize operations and strategize effectively in dynamic market environments.
Role in Statistical Studies
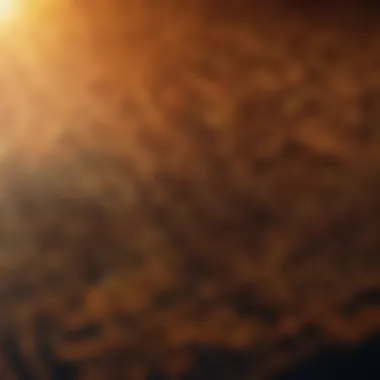
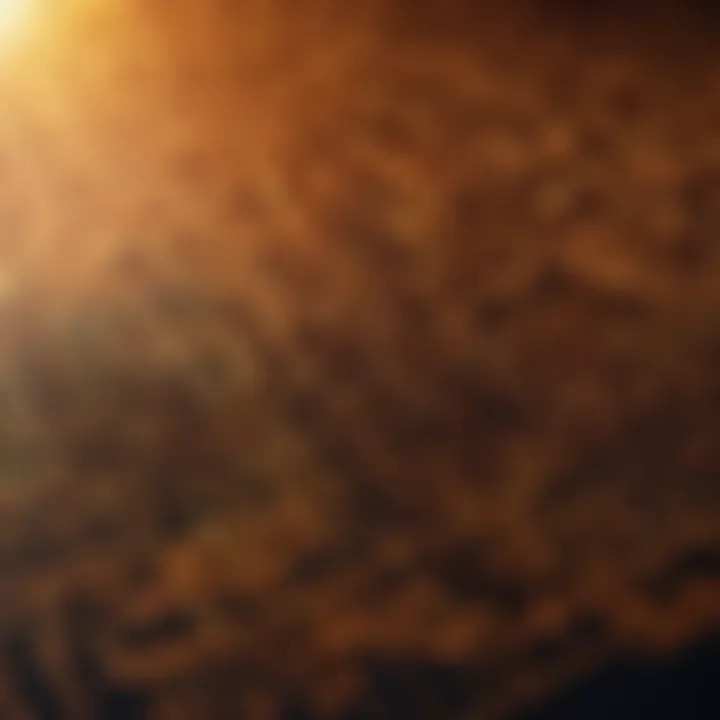
In statistical studies, random chart analysis serves as a cornerstone for exploring complex data relationships and validating hypothesis-driven research. Its role in statistical studies revolves around ensuring the integrity and reliability of data analyses conducted in research settings. The unique feature of random chart analysis in statistical studies is its ability to test assumptions and draw meaningful conclusions from empirical data. While challenges such as data quality issues may arise, the advantages of employing rigorous analytical techniques in statistical studies underscore the credibility and robustness of research findings.
Understanding Data Patterns
Exploratory Data Analysis
Identifying Trends in Random Data
Identifying trends in random data is a crucial aspect of exploratory data analysis within the realm of random chart analysis as it helps uncover underlying patterns and relationships. By identifying trends, analysts can gain insights into how variables interact and evolve over time, highlighting potential correlations and dependencies. This process aids in understanding the behavior of the data and forecasting future trends, enhancing decision-making processes and strategic planning. The key characteristic of identifying trends lies in its ability to reveal data dynamics and provide a basis for predictive modeling, making it a valuable choice for this article. However, it is essential to note that while identifying trends offers valuable insights, it also poses challenges such as data variability and complex patterns that may require sophisticated analytical tools to decipher.
Detecting Outliers
Detecting outliers plays a pivotal role in exploratory data analysis within random chart analysis by identifying data points that deviate significantly from the norm. Outliers can skew analyses and distort results, making their detection vital for obtaining accurate and reliable findings. By recognizing outliers, analysts can assess data quality, identify errors or anomalies, and make informed decisions regarding their inclusion or exclusion in subsequent analyses. The unique feature of detecting outliers lies in its ability to flag potentially influential data points that require special attention, thereby improving the robustness of analytical models. While detecting outliers enhances data integrity, it also introduces complexities such as determining the appropriate threshold for outlier detection and addressing the potential impact of outlier removal on overall analysis outcomes.
Techniques for Random Chart Analysis
In the realm of random chart analysis, understanding the different techniques plays a crucial role in deciphering the complexities embedded within the data. Techniques for Random Chart Analysis serve as the cornerstone for extracting meaningful insights and understanding underlying patterns. By employing these methodologies, analysts can unravel hidden trends and outliers that might not be evident at first glance. This section delves into the importance of Techniques for Random Chart Analysis within the context of decoding and interpreting random charts in various industries and statistical studies. It illuminates the specific elements, benefits, and considerations that shape the foundation of this analytical process.
Statistical Measures
Mean, Median, Mode
Mean, Median, and Mode stand as fundamental statistical measures that hold significance in the landscape of random chart analysis. These measures contribute by providing essential indicators of central tendency, dispersion, and the most frequently occurring value in a data set. The Mean represents the average value of a dataset, the Median showcases the middle value when the data is sorted, and the Mode reflects the value that occurs most frequently. In the context of this article, Mean, Median, and Mode offer a robust framework for understanding the distribution and characteristics of random data. Despite each measure having its strengths and limitations, they collectively aid in deriving meaningful insights from complex datasets.
Standard Deviation
Standard Deviation serves as a critical statistical measure that assesses the extent of variation or dispersion in a dataset. It highlights the average distance between each data point and the Mean, providing crucial insights into the data's volatility or stability. In the context of random chart analysis, Standard Deviation plays a pivotal role in measuring the spread of data points and identifying potential outliers. While Standard Deviation aids in quantifying the degree of uncertainty or variability within a dataset, its application within this article offers a comprehensive framework for assessing data consistency and identifying deviations from the norm.
Data Visualization Methods
Histograms
Histograms serve as powerful visualization tools that depict the frequency distribution of numerical data. This graphical representation divides data into intervals or bins, depicting the data's distribution shape and identifying trends or patterns within the dataset. In the context of random chart analysis, histograms offer a visual representation of the data's characteristics, aiding analysts in identifying outliers, clusters, and general trends. The unique feature of histograms lies in their ability to provide a concise summary of the data distribution, making them essential for exploring data patterns and extracting insights efficiently.
Scatter Plots
Scatter Plots represent data points on a two-dimensional graph, illustrating the relationship between two variables. These plots help in identifying correlations, clusters, and outliers within the data, offering valuable insights into patterns or trends that might exist. In the context of this article, scatter plots enable analysts to visualize the data points' dispersion and relationship, facilitating the identification of underlying patterns or anomalies. Their advantage lies in their simplicity and effectiveness in showcasing the data's characteristics visually, making them an indispensable tool in random chart analysis.
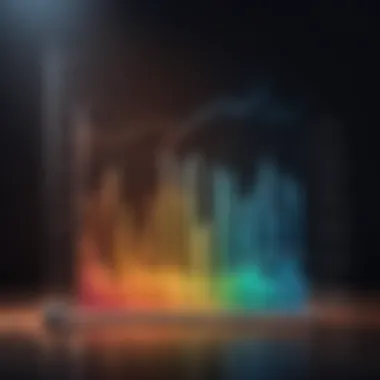
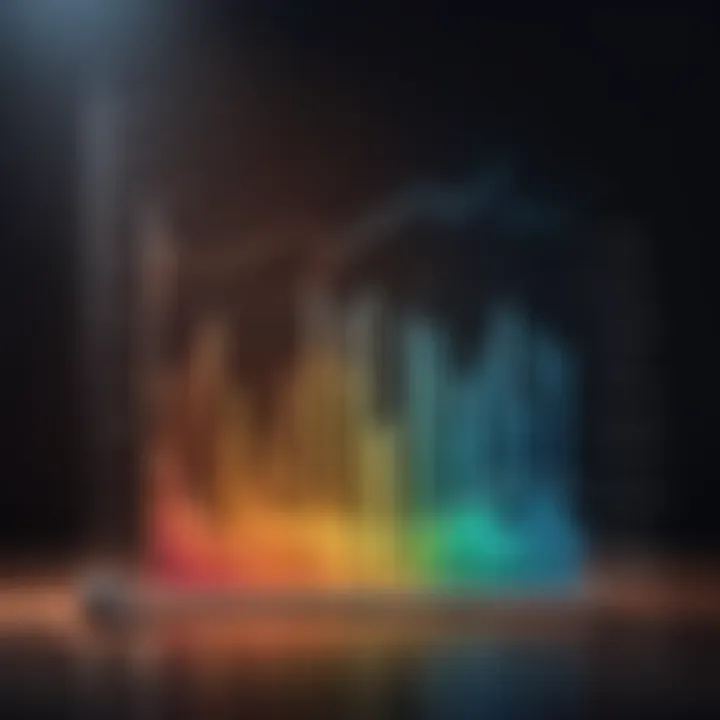
Machine Learning Models
Decision Trees
Decision Trees are structured models that aid in making decisions based on multiple conditions or attributes within a dataset. They offer a transparent representation of decision-making processes, allowing analysts to interpret and understand the factors influencing outcomes. In the realm of random chart analysis, decision trees provide a systematic approach to classify data, identify patterns, and infer meaningful insights from complex datasets. The unique feature of decision trees lies in their ability to handle both categorical and numerical data, making them versatile tools for data analysis.
Neural Networks
Neural Networks simulate the functioning of the human brain, comprising interconnected nodes that process information and learn patterns from data inputs. These networks excel in identifying complex relationships within data, enabling deep learning and pattern recognition tasks. In the context of this article, neural networks offer a sophisticated approach to analyzing random chart data, uncovering intricate patterns and trends that might elude traditional analytical methods. Despite their computational intensity, neural networks present a cutting-edge solution for tackling the challenges of random chart analysis, pushing the boundaries of data interpretation and insights.
Challenges in Random Chart Analysis
Importance of Challenges in Random Chart Analysis in this article
In the realm of random chart analysis, navigating through challenges is crucial to ensure the accuracy and reliability of data interpretation. Addressing challenges head-on not only fosters a deeper understanding of data patterns but also paves the way for extracting meaningful insights that drive decision-making processes. Within this article, the section on Challenges in Random Chart Analysis serves as a cornerstone for dissecting the complexities involved in decoding random charts, shedding light on crucial elements that shape analytical outcomes.
Overfitting and Underfitting
Balancing Model Complexity:
Balancing Model Complexity plays a pivotal role in refining the accuracy of predictive models in random chart analysis. The essence of Balancing Model Complexity lies in finding the sweet spot between a model that is too simple to capture the intricacies of the data and one that is overly complex, leading to overfitting. By striking this balance effectively, analysts can ensure that the model adapts well to unseen data while avoiding the pitfalls of underfitting or overfitting, thus enhancing the robustness of the analysis within this article.
Key to its significance is the ability to tailor the model's complexity to the specific requirements of the data set, ensuring that it captures essential patterns without becoming too rigid or too flexible. This nuanced approach to model complexity places it as a preferred choice within this article, offering a structured methodology to navigate the challenges present in random chart analysis. While the unique feature of Balancing Model Complexity lies in its adaptability to diverse data landscapes, it also presents considerations regarding the trade-offs between model sophistication and generalizability, a critical aspect for analysts within this article seeking to derive accurate insights.
Avoiding Biases:
Avoiding Biases is a critical factor in maintaining the integrity and objectivity of the analysis conducted on random charts. By actively mitigating biases, analysts can ensure that the interpretations drawn from the data are rooted in factual evidence rather than preconceived notions or skewed perspectives. This meticulous approach reshapes the narrative within this article, infusing the analysis with a sense of neutrality and impartiality that underpins the decision-making processes.
The key characteristic of Avoiding Biases is its systematic approach to identifying potential prejudices within the analysis process and implementing measures to minimize their impact. Its popularity stems from its ability to enhance the credibility of insights derived from random charts, positioning it as a fundamental aspect of analytical practices within this article. While Avoiding Biases excels in promoting transparency and accuracy, practitioners must navigate challenges related to unconscious biases and contextual influences, illustrating the nuanced nature of bias mitigation in random chart analysis.
Data Quality Issues
Missing Values:
Within the landscape of random chart analysis, grappling with Missing Values underscores the significance of data completeness and accuracy in deriving reliable insights. Missing Values present a unique challenge, requiring analysts to devise strategies that address these data gaps without compromising the integrity of the analysis. The contribution of Missing Values within this article lies in emphasizing the importance of data imputation techniques and robust handling mechanisms to bridge these gaps effectively.
A key characteristic of Missing Values is their potential to skew analytical outcomes if not addressed strategically, making it imperative for analysts within this article to implement informed decisions to handle missing data points. The beneficial aspect of Missing Values lies in highlighting the necessity of thorough data preprocessing steps to ensure data integrity and foster in-depth analysis. However, analysts must navigate the disadvantages such as increased computational complexity and potential introduction of noise during imputation, showcasing the multidimensional nature of this challenge in random chart analysis.
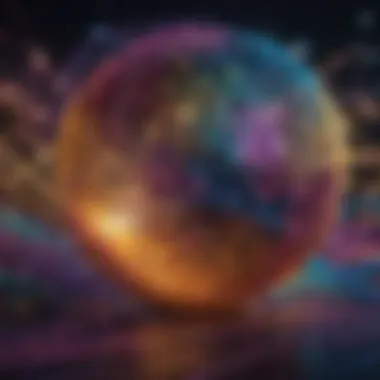
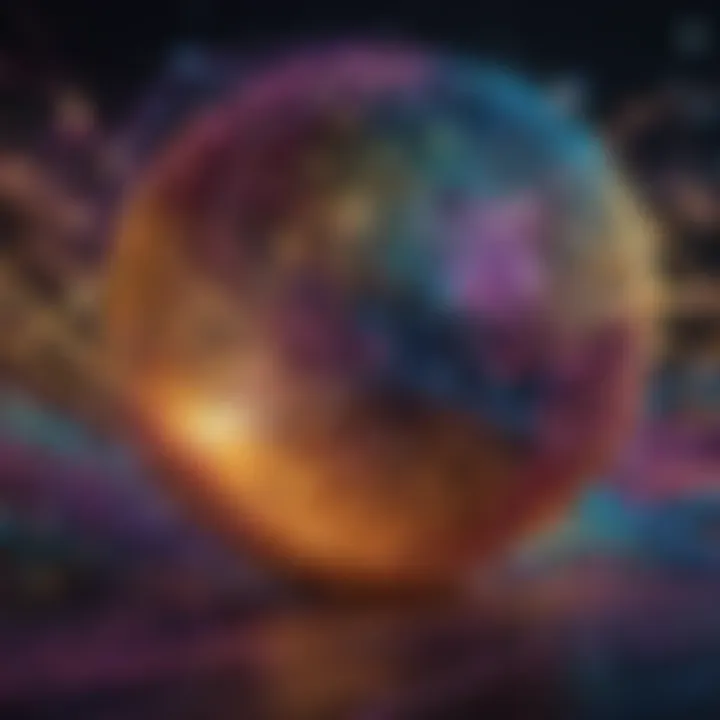
Data Imbalance:
Data Imbalance poses a significant challenge in random chart analysis, impacting the reliability and representativeness of analytical outcomes. The prevalence of Data Imbalance necessitates a nuanced approach that addresses the skewed distribution of data classes to prevent biased conclusions. Within this article, Data Imbalance surfaces as a critical consideration that shapes the methodologies employed to rectify skewed datasets and enhance the robustness of the analysis.
The key characteristic of Data Imbalance lies in its potential to influence the training of machine learning models, underscoring the need for techniques like oversampling or undersampling to address class imbalance effectively. Its popularity within this article stems from its role in fostering equitable representations of data classes, ensuring that insights derived from random charts are comprehensive and unbiased. While Data Imbalance offers advantages in promoting fair analysis practices, challenges such as increased model complexity and potential loss of information present considerations for analysts aiming to strike a balance between data equity and analytical accuracy.
Interpreting Results
Drawing Valid Conclusions:
Drawing Valid Conclusions in the context of random chart analysis is paramount to deriving actionable insights that drive informed decision-making processes. The ability to draw valid conclusions hinges on the meticulous examination and interpretation of data patterns, ensuring that analytical outcomes are rooted in evidence-based reasoning rather than conjecture. Within this article, Drawing Valid Conclusions emerges as a guiding principle that shapes the narrative of data interpretation, offering a structured approach to extracting meaningful insights from random charts.
The key characteristic of Drawing Valid Conclusions lies in its emphasis on thorough data analysis techniques and logical deductions that substantiate the conclusions drawn. Its popularity within this article arises from its role in fostering data-driven decision-making, thereby instilling confidence in the analytical outputs derived from random charts. While Drawing Valid Conclusions enhances the credibility of analysis, considerations regarding subjectivity in interpretation and the need for robust validation mechanisms highlight the complexities inherent in drawing sound conclusions within the realm of random chart analysis.
Assessing Statistical Significance:
Assessing Statistical Significance serves as a beacon of validation in random chart analysis, enabling analysts to gauge the reliability and relevance of analytical findings against established statistical thresholds. This critical evaluation ensures that the insights derived from random charts are not mere coincidences but rather meaningful patterns backed by statistical rigor. Framed within this article, Assessing Statistical Significance acts as a compass that guides analysts towards confident decision-making based on solid statistical grounds.
The key characteristic of Assessing Statistical Significance is its role in quantifying the likelihood of observed results occurring by chance, offering a quantitative lens through which analytical outcomes can be evaluated. Its benevolent choice for this article manifests in its capacity to instill confidence in the reliability of analytical insights, fostering a culture of evidence-based decision-making. While Assessing Statistical Significance underscores the importance of statistical verification, challenges such as selecting appropriate significance levels and guarding against type I and type II errors underscore the complexities involved in ascertaining statistical relevance in random chart analysis.
Future Trends in Random Chart Analysis
In this article, we delve into the future trends shaping the landscape of random chart analysis, a critical aspect for understanding the evolving nature of data patterns and statistical inference. The exploration of future trends in this domain is paramount as it offers valuable insights into the direction and potential advancements in data analytics. By focusing on upcoming developments, we equip ourselves with the knowledge necessary to stay ahead of the curve in deciphering and interpreting random charts.
Advancements in Data Analytics
Big Data Integration
Big Data Integration stands out as a pivotal aspect of the future trends in random chart analysis. The seamless incorporation of vast and diverse datasets from various sources enhances the depth and breadth of analysis, enabling more robust insights and robust decision-making processes. One key characteristic of Big Data Integration is its ability to handle high-volume, high-velocity, and high-variety data at scale, which significantly impacts the accuracy and reliability of analytical outcomes. This choice proves beneficial in our article as it allows for a comprehensive examination of random charts with a broad data spectrum. The unique feature of Big Data Integration lies in its utilization of advanced algorithms and processing techniques to streamline data integration, offering advantages in scalability and real-time analytics. However, challenges such as data security and privacy concerns may arise, emphasizing the need for stringent measures to safeguard sensitive information.
Predictive Analytics
Predictive Analytics emerges as a key driver of future trends in random chart analysis, paving the way for predictive modeling, trend forecasting, and risk assessment. The essence of Predictive Analytics lies in its proactive approach to anticipate future outcomes based on historical data patterns and statistical algorithms. This choice gains significance in our article as it enables us to foresee potential trends and patterns in random charts, enhancing decision-making and strategy formulation. The unique feature of Predictive Analytics is its emphasis on leveraging machine learning and data mining techniques to extract actionable insights for improved predictive accuracy. While advantageous in forecasting and risk mitigation, challenges such as data complexity and model interpretation may pose hurdles in the application of Predictive Analytics.
Emerging Technologies
AI and Machine Learning
AI and Machine Learning revolutionize the future trajectory of random chart analysis by fostering automation, pattern recognition, and cognitive computing capabilities. The key characteristic of AI and Machine Learning is their ability to streamline data analysis processes, identify intricate patterns, and optimize decision-making based on statistical algorithms. This choice proves beneficial in our article as it accelerates the identification of hidden trends and outliers in random charts, enabling efficient data interpretation. The unique feature of AI and Machine Learning lies in their adaptability to evolving datasets and their capacity to enhance analytical precision through iterative learning mechanisms. Despite their advantages in predictive modeling and trend analysis, challenges like algorithm bias and model complexity require careful consideration in implementing AI and Machine Learning in random chart analysis.
Blockchain Applications
Blockchain Applications emerge as a disruptive force in the realm of random chart analysis, introducing enhanced transparency, immutability, and decentralization to data processing and validation. The key characteristic of Blockchain Applications is their decentralized ledger system, which ensures data integrity and eliminates the risk of tampering or manipulation. This choice is advantageous in our article as it guarantees the authenticity and verifiability of random chart data, fostering trust and reliability in analytical outcomes. The unique feature of Blockchain Applications lies in their smart contract functionalities, which automate data verification and validation processes, offering superior efficiency and security. However, challenges such as scalability and regulatory compliance may pose constraints on the widespread adoption of Blockchain Applications in random chart analysis.