Unveiling the Intrinsic Harmony: CFA and Machine Learning Convergence
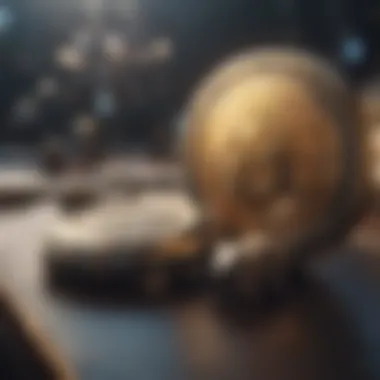
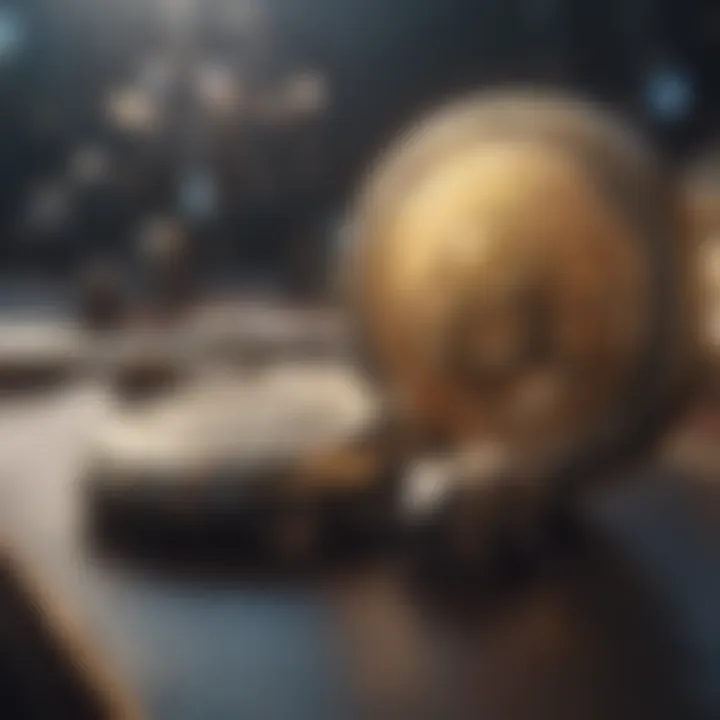
What is cfa machine learning:
The concept of CFA (Chartered Financial Analyst) machine learning is a relatively new development within the field of finance. It represents the integration of traditional CFA principles with advanced machine learning techniques. The fusion aims to leverage the capabilities of artificial intelligence in enhancing decision-making processes and data analysis within the financial sector.
CFA machine learning does not have a single creator or entity that can be attributed to its inception. Rather, it has evolved through the collaborative efforts of finance professionals and data scientists seeking to improve traditional financial analysis methods.
While the specific number of CFA machine learning implementations may not be quantifiable due to the diversity of applications and interpretations, various financial institutions and research organizations have been actively exploring and adopting machine learning techniques in conjunction with CFA principles.
The primary purpose of CFA machine learning is to enhance the accuracy, efficiency, and effectiveness of financial analyses. By integrating machine learning algorithms with CFA knowledge and principles, analysts can gain deeper insights into market trends, risk management, investment strategies, and portfolio optimization.
CFA machine learning is utilized across a wide range of financial activities, including predictive modeling, algorithmic trading, risk assessment, fraud detection, quantitative analysis, and asset valuation. Its application extends to diverse sectors such as banking, asset management, insurance, and investment firms, where data-driven decision-making is critical.
The tokenomics of CFA machine learning are dependent on various tokens that facilitate the execution of machine learning algorithms, data processing, model training, and result interpretation. These tokens may include data tokens, utility tokens, security tokens, and reward tokens, each playing a specific role in the ecosystem.
In addition to tokens, the CFA machine learning ecosystem incorporates a variety of tools and technologies to support its operations. These tools may encompass data visualization platforms, cloud computing resources, programming languages (such as Python and R), machine learning libraries (like TensorFlow and scikit-learn), and trading software for algorithmic execution.
When considering whether to swap CFA machine learning tokens as opposed to traditional trading, it is essential to understand the underlying difference between the two processes. Swapping involves exchanging one type of digital asset (such as CFA machine learning tokens) for another, typically on decentralized exchanges or swapping platforms, while trading involves buying and selling assets for fiat currency or other cryptocurrencies on centralized exchanges.
To buy CFA machine learning tokens, individuals can follow a few simple steps, including setting up a digital wallet compatible with the tokens, choosing a reputable exchange platform that lists CFA machine learning tokens, creating an account, completing KYC verification if required, depositing funds into the account, selecting the desired amount of tokens, executing the purchase order, and storing the tokens securely in the wallet.
Overall, the integration of CFA principles with machine learning algorithms represents a groundbreaking initiative in the financial domain, paving the way for enhanced decision-making processes, improved analytical capabilities, and greater insights into market dynamics.
Introduction to CFA and Machine Learning
Chartered Financial Analyst (CFA) and Machine Learning intersect at a fascinating crossroads of traditional financial expertise and cutting-edge technological advancements. In this article, we delve into the importance of understanding how these two domains converge, providing a unique perspective on the integration of quantitative analysis and data-driven decision-making processes. The synergy between CFA principles and machine learning techniques opens new avenues for financial professionals seeking to enhance their analytical capabilities and stay ahead in a rapidly evolving landscape.
Understanding Chartered Financial Analyst (CFA)
CFA Qualifications and Expertise
When exploring CFA Qualifications and Expertise, we unveil a sophisticated framework that equips professionals with in-depth knowledge of investment management, financial analysis, and ethical best practices. The rigorous curriculum and examination process of the CFA program validate individuals' expertise in areas such as portfolio management, equities, fixed income, and ethics. This specialized skill set not only enhances one's credibility in the financial industry but also demonstrates a commitment to continuous learning and professional development.
CFA Code of Ethics and Standards
The CFA Code of Ethics and Standards embodies the highest principles of integrity, competence, and diligence within the financial sector. By adhering to these ethical guidelines, CFAs pledge to prioritize clients' interests, uphold confidentiality, and act with professionalism and integrity at all times, fostering trust and credibility in their professional relationships. This ethical compass not only distinguishes CFAs as trustworthy professionals but also serves as a guiding light in navigating complex ethical dilemmas and regulatory requirements.
Relevance of CFA in Financial Analysis
The relevance of CFA in financial analysis lies in its emphasis on thorough research, critical analysis, and risk management, aligning with the core principles of sound investment decisions. CFAs play a vital role in deciphering complex financial data, evaluating investment opportunities, and constructing robust financial models to drive informed decision-making processes. Their expertise in financial statement analysis, asset valuation, and risk assessment equips them with the tools to navigate dynamic market conditions and deliver value-added insights to stakeholders.
Overview of Machine Learning
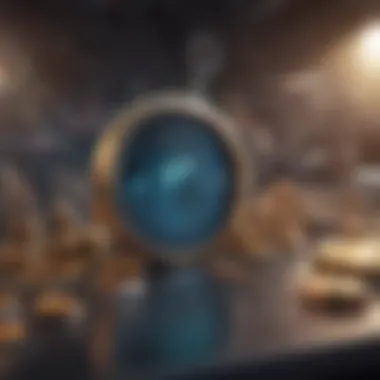
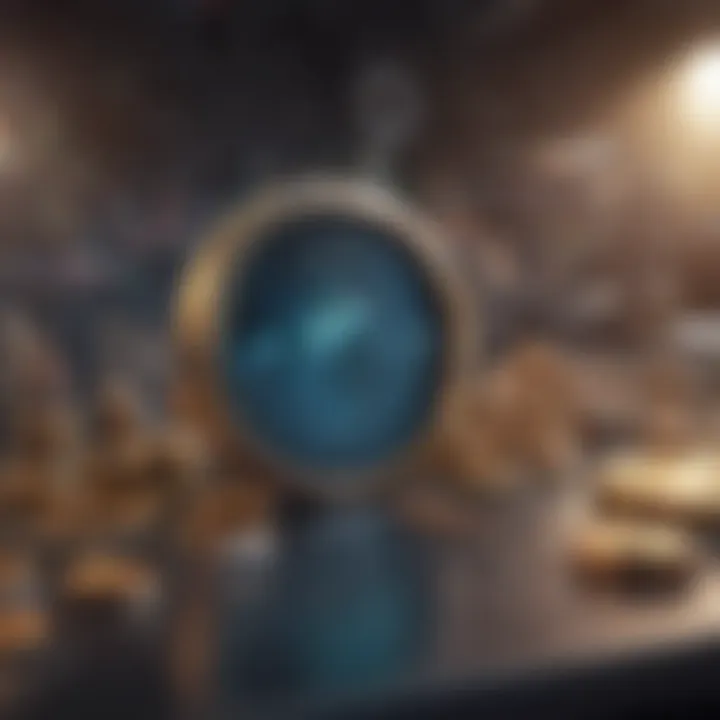
Definition and Applications
Machine Learning, a subset of artificial intelligence, empowers algorithms to learn from data, recognize patterns, and make predictions without explicit programming instructions. In the context of finance, Machine Learning finds applications in fraud detection, credit scoring, algorithmic trading, and risk management, revolutionizing traditional operational processes and enhancing decision-making capabilities. Its ability to process vast amounts of data swiftly and identify intricate relationships enables financial institutions to extract valuable insights and improve operational efficiencies.
Types of Machine Learning Algorithms
Machine Learning Algorithms encompass a diverse range of models, including supervised learning, unsupervised learning, and reinforcement learning, each tailored to address specific analytical challenges. Supervised learning aids in predicting outcomes based on labeled data, while unsupervised learning uncovers patterns in unlabeled data sets, presenting new perspectives for analysis. Reinforcement learning, on the other hand, explores decision-making processes through interactions with an environment, optimizing strategies over time to achieve desired objectives.
Impact of Machine Learning in Finance
The Impact of Machine Learning in finance transcends conventional boundaries by augmenting decision-making processes, automating routine tasks, and identifying market trends with greater precision. Machine Learning empowers financial institutions to mitigate risks, customize investment strategies, and enhance customer experiences through personalized services and tailored recommendations. By leveraging advanced algorithms and predictive analytics, Machine Learning propels the finance industry towards a data-driven future, where agility and adaptability are key to sustaining competitive advantages.
Significance of Integration
Enhancing Financial Decision Making
Integrating CFA expertise with Machine Learning capabilities enhances financial decision-making by enabling professionals to leverage data analytics, quantitative models, and predictive algorithms to optimize investment strategies, identify market opportunities, and mitigate risks swiftly. This fusion of domain knowledge and technological innovation equips financial professionals with a comprehensive toolkit to navigate volatile markets and capitalize on emerging trends effectively.
Improving Risk Management Processes
The integration of Machine Learning in risk management processes revolutionizes the way financial institutions assess and mitigate risks, providing real-time insights into market dynamics, counterparty risks, and macroeconomic factors. By utilizing predictive modeling, anomaly detection, and scenario analysis, organizations can proactively identify potential risks, allocate capital efficiently, and safeguard assets against unforeseen events, ensuring business continuity and resilience in a rapidly changing environment.
Optimizing Portfolio Management
Optimizing Portfolio Management with Machine Learning algorithms streamlines asset allocation, rebalancing strategies, and performance monitoring, enhancing portfolio efficiency and maximizing returns for investors. By employing predictive analytics, sentiment analysis, and algorithmic trading models, portfolio managers can make informed investment decisions, diversify risk exposure, and capitalize on market inefficiencies, delivering superior outcomes for clients and stakeholders.
Applications of Machine Learning in Financial Analysis
In this section of the article, we delve into the crucial role of machine learning in enhancing financial analysis processes. Machine learning applications have significantly transformed how financial data is analyzed and interpreted, revolutionizing decision-making in the field. By leveraging advanced algorithms and data processing capabilities, machine learning empowers professionals to extract valuable insights from complex datasets, ultimately optimizing strategies and outcomes in the financial domain.
Predictive Modeling
Stock Price Forecasting
Stock price forecasting is a fundamental aspect of financial analysis that utilizes historical data, market trends, and various indicators to predict future stock price movements. In the context of this article, stock price forecasting illustrates the predictive power of machine learning in generating actionable insights for investors and analysts. The key characteristic of stock price forecasting lies in its ability to identify patterns and trends within financial data, aiding stakeholders in making informed investment decisions. While stock price forecasting offers valuable predictive capabilities, it is essential to acknowledge its limitations, such as sensitivity to market volatility and unforeseen events that may impact stock prices.
Credit Risk Assessment
Credit risk assessment plays a critical role in evaluating the creditworthiness of individuals or entities, helping to mitigate financial risks for lenders and investors. Within the scope of this article, credit risk assessment showcases how machine learning algorithms can streamline the risk evaluation process, enhancing accuracy and efficiency. The unique feature of credit risk assessment lies in its ability to analyze vast amounts of data to assess borrowers' credit profiles and predict default probabilities. Despite its advantages in improving risk management practices, credit risk assessment may face challenges related to model bias and data quality issues.
Market Trend Analysis
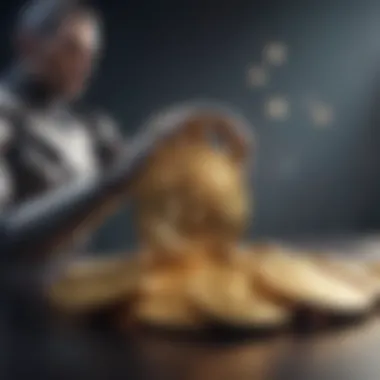
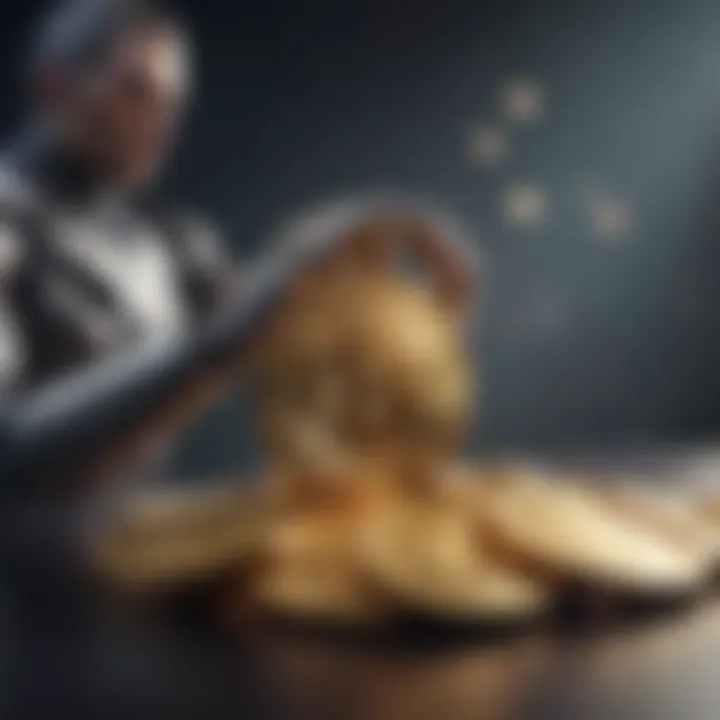
Market trend analysis involves studying historical market data and identifying patterns or trends that can influence future market movements. In the context of this article, market trend analysis highlights the significance of utilizing machine learning techniques to uncover valuable insights from market data. The distinctive feature of market trend analysis lies in its capacity to detect emerging trends and market shifts, empowering stakeholders to make strategic decisions based on data-driven analysis. While market trend analysis provides valuable intelligence for financial professionals, it is essential to consider factors like model interpretation and data accuracy to ensure reliable forecasting outcomes.
Quantitative Trading Strategies
Quantitative trading strategies are algorithm-based approaches to trading financial instruments, leveraging mathematical models and computational techniques to execute trades. This section explores how machine learning enhances quantitative trading strategies, enabling faster decision-making and execution processes in the financial markets. Algorithmic trading stands out for its ability to automate trading decisions based on predefined rules and conditions, offering efficiency and consistency in trading operations. Despite its advantages, algorithmic trading also faces challenges such as system glitches and algorithmic biases that may impact trading outcomes.
High-Frequency Trading
High-frequency trading involves executing a large number of trades at rapid speeds, capitalizing on small price differentials in the market. In the context of this article, high-frequency trading exemplifies the intersection of machine learning and finance, showcasing how advanced algorithms and technologies drive rapid trading activities. The key characteristic of high-frequency trading lies in its focus on speed and volume, enabling market participants to capitalize on microsecond opportunities. While high-frequency trading offers liquidity and market efficiency benefits, it is essential to address concerns regarding market manipulation and stability risks.
Sentiment Analysis
Sentiment analysis is a technique that involves analyzing textual data to gauge public sentiment or opinions about specific assets or markets. Within the realm of this article, sentiment analysis demonstrates how machine learning algorithms can extract actionable insights from social media, news articles, and other sources to inform trading decisions. The unique feature of sentiment analysis lies in its ability to quantify qualitative data and sentiment trends, providing traders with additional indicators for market analysis. Despite its advantages in understanding market sentiment, sentiment analysis may encounter challenges related to data accuracy and context interpretation.
Risk Management Techniques
Risk management techniques play a vital role in safeguarding financial institutions and investors against potential threats and uncertainties in the market. This section explores how machine learning tools enhance risk management practices by enabling proactive risk identification and mitigation strategies. VaR modeling, a quantitative method for estimating financial risk, offers valuable insights into potential losses under specified confidence levels and timeframes. While VaR modeling aids in risk assessment and decision-making, it is essential to recognize its limitations, such as assumptions about market conditions and the potential for model inaccuracies.
Stress Testing
Stress testing involves analyzing the impact of adverse events or scenarios on financial institutions or investment portfolios. In the context of this article, stress testing exemplifies how machine learning algorithms simulate scenarios to assess the resilience of financial systems and assets. The key characteristic of stress testing lies in its ability to quantify potential losses under extreme conditions, providing valuable risk insights to stakeholders. Despite its benefits in enhancing financial resilience, stress testing may face challenges related to scenario design complexity and data model calibration.
Fraud Detection
Fraud detection is a crucial aspect of risk management, aiming to identify and prevent fraudulent activities within financial systems. Within the scope of this article, fraud detection showcases how machine learning tools enhance fraud detection capabilities by analyzing transaction patterns, user behavior, and other indicators of potential fraud. The unique feature of fraud detection lies in its adaptive algorithms that continuously learn and adapt to new fraud schemes, improving detection rates over time. Despite its advantages in fraud prevention, fraud detection systems may encounter challenges such as false positives and model interpretability issues.
Challenges and Opportunities for CFAs
In the realm of financial analysis, the challenges and opportunities for Chartered Financial Analysts (CFAs) play a pivotal role in shaping the landscape of modern finance. These challenges not only test the expertise of CFAs but also present unique opportunities for growth and innovation within the industry. CFAs face the ongoing challenge of staying abreast of rapidly evolving market trends, regulations, and technological advancements. This necessitates a continual process of upskilling and adaptation to remain competitive in a dynamic financial environment.
The opportunities for CFAs lie in their ability to leverage advanced tools such as machine learning to enhance decision-making processes and analytical capabilities. By embracing machine learning techniques, CFAs can gain deeper insights into market behaviors, improve risk management strategies, and optimize portfolio performance. Furthermore, the integration of machine learning with traditional financial analysis methods opens doors to innovative approaches that can streamline operations and unlock new possibilities for generating alpha.
Skill Enhancement Requirements
Programming Proficiency
Programming proficiency stands as a fundamental skill enhancement requirement for CFAs looking to navigate the intersection of finance and technology effectively. The ability to code empowers CFAs to develop customized algorithms, conduct in-depth data analysis, and automate repetitive tasks, thus enhancing efficiency and accuracy in their analyses. Proficiency in programming languages like Python, R, and SQL is particularly beneficial for CFAs working with machine learning models as these languages offer robust libraries and frameworks tailored for financial data analysis.
Statistical Analysis Skills
Statistical analysis skills are indispensable for CFAs aiming to derive meaningful insights from complex financial datasets. Proficiency in statistical methods allows CFAs to interpret historical data trends, forecast future market movements, and quantify risk exposures accurately. By leveraging statistical analysis skills, CFAs can build sophisticated models to support investment decision-making, risk management, and performance evaluation with a high degree of precision.
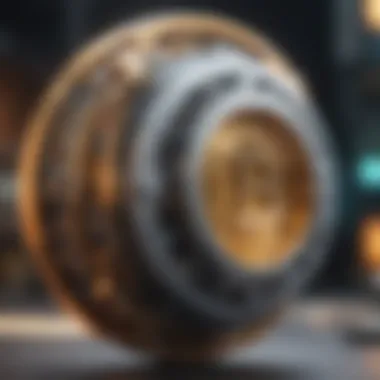
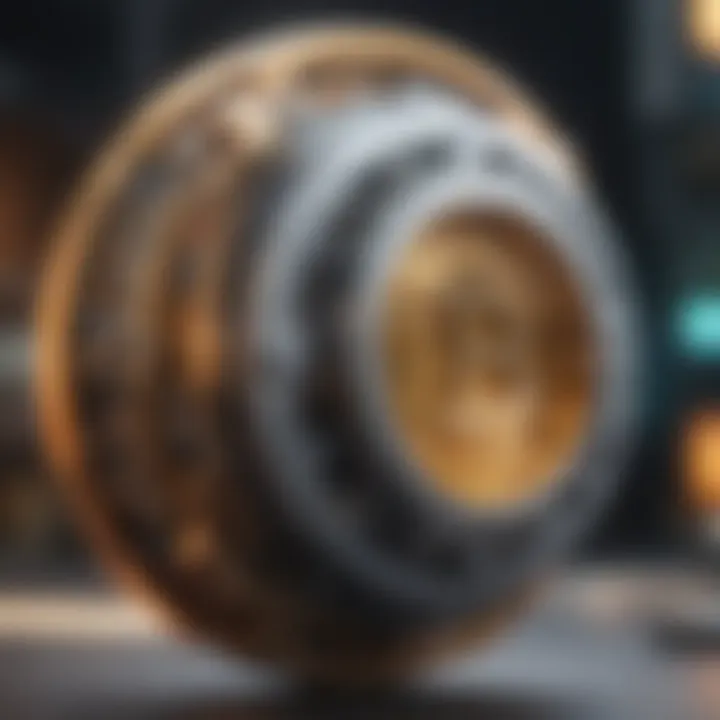
Interdisciplinary Knowledge
Interdisciplinary knowledge acts as a catalyst for CFAs seeking to expand their expertise beyond traditional financial paradigms. By integrating insights from disciplines such as data science, behavioral economics, and computer science, CFAs can gain a holistic perspective on market dynamics and investor behavior. This interdisciplinary approach equips CFAs with the versatility to tackle complex financial challenges from multifaceted angles, fostering innovation and adaptability in an ever-changing financial landscape.
Ethical Considerations
Data Privacy Issues
Data privacy issues emerge as a critical consideration for CFAs operating at the intersection of finance and machine learning. The proliferation of sensitive financial data necessitates stringent data protection measures to safeguard client information and maintain regulatory compliance. CFAs must prioritize data privacy by implementing robust encryption protocols, access controls, and data anonymization techniques to mitigate the risks of unauthorized access or data breaches.
Bias and Fairness Concerns
Bias and fairness concerns loom large in the realm of algorithmic decision-making, raising ethical dilemmas for CFAs leveraging machine learning tools. The inherent biases present in training datasets and algorithmic models can lead to discriminatory outcomes in financial analyses, impacting investment decisions and market perceptions. CFAs must address bias and fairness concerns by conducting regular audits of their models, incorporating fairness metrics, and promoting transparency in their decision-making processes to uphold ethical standards.
Regulatory Compliance
Regulatory compliance stands as a cornerstone of ethical conduct for CFAs embracing machine learning technologies in finance. Adhering to stringent regulatory frameworks such as GDPR, SEC regulations, and industry standards is paramount to ensure the fair and legal use of AI-powered tools in financial analysis. CFAs bear the responsibility of aligning their practices with regulatory guidelines, disclosing the use of machine learning algorithms transparently, and upholding ethical standards to protect investor interests and maintain trust in the financial markets.
Future Prospects
Emerging Trends in Financial Technology
The emergence of cutting-edge technologies such as blockchain, quantum computing, and decentralized finance (DeFi) presents unparalleled opportunities for CFAs to adapt and innovate in the digital age. By staying abreast of emerging trends in financial technology, CFAs can leverage disruptive solutions to enhance their analytical capabilities, streamline operations, and gain a competitive edge in the increasingly digitized financial landscape. Embracing innovations like blockchain-based smart contracts, AI-driven analytics, and algorithmic trading platforms can revolutionize traditional financial practices and open doors to new revenue streams for CFAs.
Continuous Learning Imperative
The rapid pace of technological advancements demands a culture of continuous learning and skill development for CFAs seeking to thrive in a tech-driven financial ecosystem. Embracing a mindset of lifelong learning enables CFAs to stay ahead of industry trends, absorb new knowledge, and adapt to evolving market dynamics effectively. By investing in professional development programs, attending industry conferences, and pursuing certifications in emerging technologies, CFAs can sharpen their skill sets, expand their networks, and position themselves as thought leaders in the ever-changing financial landscape.
Adaptation to Technological Disruption
Adapting to technological disruption is paramount for CFAs to navigate the shifting currents of the digital economy successfully. The ability to embrace innovative technologies, such as machine learning, artificial intelligence, and big data analytics, allows CFAs to drive efficiency gains, identify new business opportunities, and deliver enhanced value to clients. By proactively assessing tech disruptions, experimenting with novel solutions, and fostering a culture of innovation within their organizations, CFAs can future-proof their careers and capitalize on the transformative power of technology in finance.
Conclusion
The Synergy Unleashed
Unleashing Potential with CFA and Integration
Unleashing Potential with CFA and ML Integration plays a crucial role in enhancing the overall financial landscape. This aspect focuses on leveraging the analytical proficiency of CFAs with the predictive power of machine learning algorithms. The key characteristic of this integration lies in its ability to combine human expertise with computational accuracy, ensuring more precise and efficient decision-making in complex financial scenarios. While the unique feature of this synergy offers unprecedented insights and foresight into market dynamics, its primary advantage lies in providing a multidimensional approach to financial analysis. By seamlessly blending qualitative insights with quantitative models, the Unleashing Potential with CFA and ML Integration facilitates a holistic perspective, enabling professionals to make well-informed and strategic decisions.
The Path Ahead for Financial Professionals
The Path Ahead for Financial Professionals delves into the evolving role of professionals in embracing technological advancements. This aspect underscores the importance of continuous learning and adaptation to navigate the digital transformation within the financial industry. The key characteristic here is the emphasis on acquiring new skill sets, such as data analytics and programming, to meet the demands of a data-driven environment. By recognizing the imperative need for upskilling and staying abreast of emerging technologies, financial professionals can stay competitive and relevant in a dynamic market landscape. The unique feature of The Path Ahead for Financial Professionals lies in its forward-thinking approach, preparing individuals to seize opportunities and tackle challenges presented by technological disruptions. While offering avenues for growth and innovation, this aspect also poses the challenge of rapid adaptability and resilience in an ever-changing digital era.
Seizing Opportunities in the Digital Era
Seizing Opportunities in the Digital Era encapsulates the proactive stance that professionals need to take to leverage technological advancements effectively. This aspect highlights the critical need for embracing innovation and exploring new avenues for value creation in a digital-centric world. The key characteristic of seizing opportunities lies in the agility and flexibility required to capitalize on emerging trends and technologies. By incorporating cutting-edge tools and methodologies into financial practices, professionals can stay ahead of the curve and unlock potentials for growth and development. The unique feature of Seizing Opportunities in the Digital Era lies in its ability to cultivate a culture of innovation and adaptability, fostering a mindset geared towards continuous improvement and advancement. While presenting numerous advantages in terms of efficiency and competitiveness, this aspect also underscores the importance of managing risks and addressing ethical considerations in an increasingly digitized financial landscape.