Unveiling the Fascinating Potential of Synaptic AI in Modern Technology
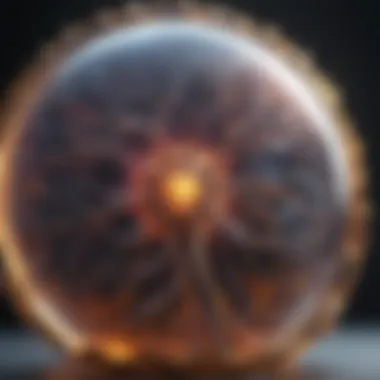
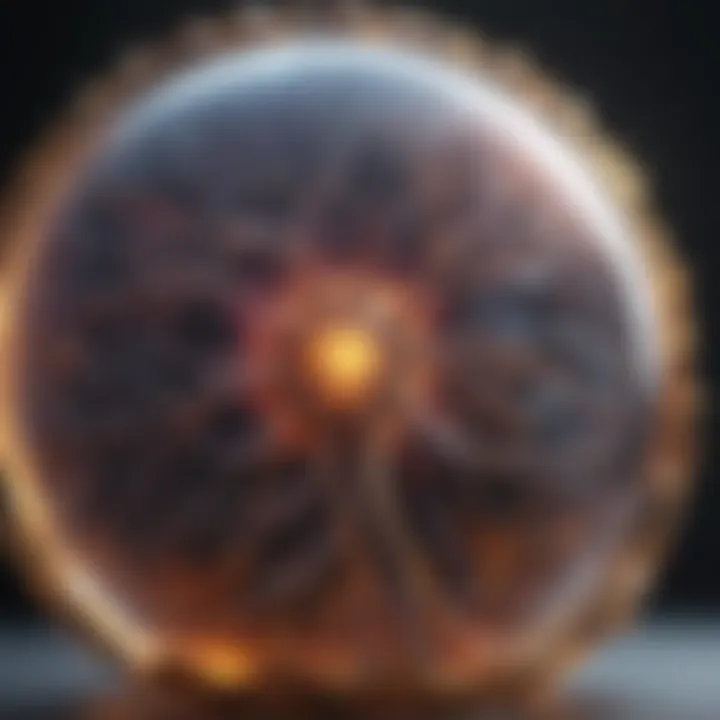
What is synaptic AI:
Synaptic AI, a revolutionary concept in the realm of artificial intelligence, is the brainchild of a team of visionary tech innovators looking to push the boundaries of machine learning. Leveraging intricate neural network models, Synaptic AI aims to mimic the human brain's synaptic connections to enhance computing capabilities.
Currently, there exist several iterations of Synaptic AI, each evolving to improve complex data processing and decision-making. Its iterations are tailored for specific applications, ranging from image recognition to natural language processing, offering diversified solutions across the technological spectrum.
The core purpose of Synaptic AI lies in enhancing machine learning algorithms by simulating biological synapses, enabling more efficient and dynamic neural computations. By bridging the gap between artificial and biological intelligence, Synaptic AI opens doors to unprecedented possibilities in autonomous systems, healthcare diagnostics, and financial predictions.
Professionals and developers alike utilize Synaptic AI for a myriad of tasks, including predicting customer behavior patterns, optimizing supply chain logistics, and personalizing user experiences. Its versatility and adaptability make it a coveted tool in various industries seeking data-driven insights and predictive analytics.
The Synaptic AI tokenomics are intricately tied to specific tokens that govern the platform's incentivization mechanisms, such as utility tokens facilitating network transactions, reward tokens for contributors, and governance tokens for community voting.
Within the expansive Synaptic AI ecosystem, users can harness a diverse array of tools, including data visualization platforms, diagnostic modules, and predictive analytics dashboards. This rich toolset empowers users to streamline workflows, unearth actionable insights, and drive informed decision-making.
When it comes to exchanging Synaptic AI, users engage in swapping tokens rather than traditional trading. The distinction lies in the decentralized nature of token exchanges, offering a more peer-to-peer and secure alternative to centralized trading platforms. Swapping Synaptic AI tokens involves direct token-to-token swaps, ensuring swift and efficient transactions without the need for intermediaries.
To acquire Synaptic AI, interested parties can navigate user-friendly decentralized exchanges and crypto platforms supporting the token. By following simple steps like creating a digital wallet, depositing funds, and executing the swap, users can swiftly add Synaptic AI tokens to their digital asset portfolio, ready to leverage its powerful machine learning capabilities for various applications.
Introduction
In this groundbreaking article, we embark on a journey to delve deep into the revolutionary realm of synaptic AI. Synaptic AI represents the convergence of neuroscience and artificial intelligence, paving the way for a new era of technological advancement. This Introduction sets the stage for a comprehensive exploration of the intricacies, capabilities, and implications of Synaptic AI within the modern technological landscape. By dissecting the emergence, significance, and functioning of Synaptic AI, readers will be equipped with a profound understanding of this cutting-edge technology.
Emergence of Synaptic AI
The fusion of neuroscience and artificial intelligence
The fusion of neuroscience and artificial intelligence is a pivotal development within the realm of Synaptic AI. It signifies the amalgamation of biological insights with computational power, leveraging the principles of brain function to enhance AI systems. This amalgamation enriches AI models with a deep understanding of neural mechanisms, enabling more sophisticated learning and decision-making processes. The fusion of neuroscience and artificial intelligence is instrumental in replicating human-like cognitive abilities in AI systems, leading to significant advancements in various domains.
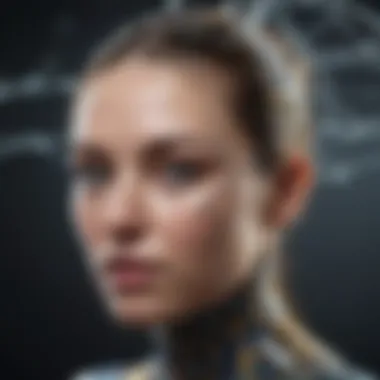
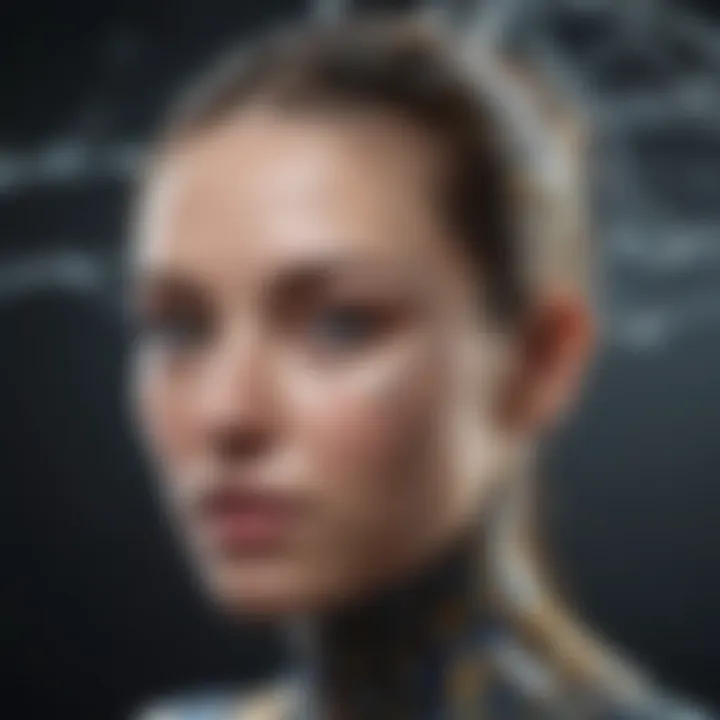
Replicating human synaptic connections
Replicating human synaptic connections lies at the core of Synaptic AI, mimicking the intricate network of synapses in the human brain. This replication harnesses the power of synaptic plasticity to foster adaptive learning and memory processes within AI systems. By emulating the dynamic nature of synaptic connections, AI algorithms can continuously evolve and enhance their performance over time. The replication of human synaptic connections in Synaptic AI contributes to unprecedented learning capabilities and cognitive adaptability, propelling AI systems towards greater efficiency and versatility.
Significance of Synaptic AI
Enhanced learning capabilities
Synaptic AI's enhanced learning capabilities redefine the landscape of artificial intelligence by introducing immersive learning mechanisms inspired by the human brain. This innovation allows AI systems to grasp intricate patterns, make informed decisions, and adapt to evolving scenarios with remarkable agility. The enhanced learning capabilities of Synaptic AI not only accelerate the pace of knowledge acquisition but also empower machines to navigate complex tasks with superior proficiency.
Adaptability to dynamic environments
The adaptability of Synaptic AI to dynamic environments underscores its resilience and versatility in real-world scenarios. Unlike traditional AI systems that operate within predefined parameters, Synaptic AI thrives on uncertainty and variability, thriving in dynamic settings that demand quick decision-making and agile responses. This adaptability equips AI systems with the capacity to learn from diverse environmental cues, adjust their strategies accordingly, and excel in ever-changing conditions, making them indispensable assets in a rapidly evolving technological landscape.
Fundamentals of Synaptic AI
Synaptic AI serves as the backbone of modern technological advancements, playing a pivotal role in revolutionizing artificial intelligence capabilities. Understanding the fundamentals of synaptic AI is crucial for grasping its profound impact on various industries and fields. In this article, we will dissect the core elements of synaptic AI, shedding light on its intricate mechanisms and applications.
Neural Networks
Neuron simulation in AI
Neuron simulation in AI is a fundamental aspect of synaptic AI that mimics the workings of neurons in the human brain. This simulation allows AI systems to process information and make decisions through interconnected nodes, resembling the functions of biological neurons. The key characteristic of neuron simulation lies in its ability to perform complex computations and learning tasks, making it a popular choice in developing sophisticated AI algorithms. The unique feature of neuron simulation is its adaptability and learning capabilities, enabling AI models to improve their performance over time. While advantageous in enhancing AI cognition, neuron simulation may pose challenges in terms of computational intensity and training requirements.
Synaptic weight adjustment
Synaptic weight adjustment is a critical mechanism in synaptic AI that regulates the strength of connections between artificial neurons. This adjustment process optimizes the flow of information within neural networks, refining their efficiency and accuracy. The key characteristic of synaptic weight adjustment lies in its ability to fine-tune neural connections based on training data, improving AI performance in specific tasks. This feature is beneficial for optimizing AI model outputs and learning outcomes, making it a valuable choice for enhancing neural network functionalities. However, the intricate nature of synaptic weight adjustment may involve complexities in implementation and algorithmic design, requiring careful calibration and tuning.
Learning Mechanisms
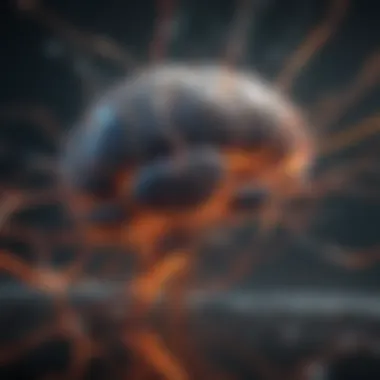
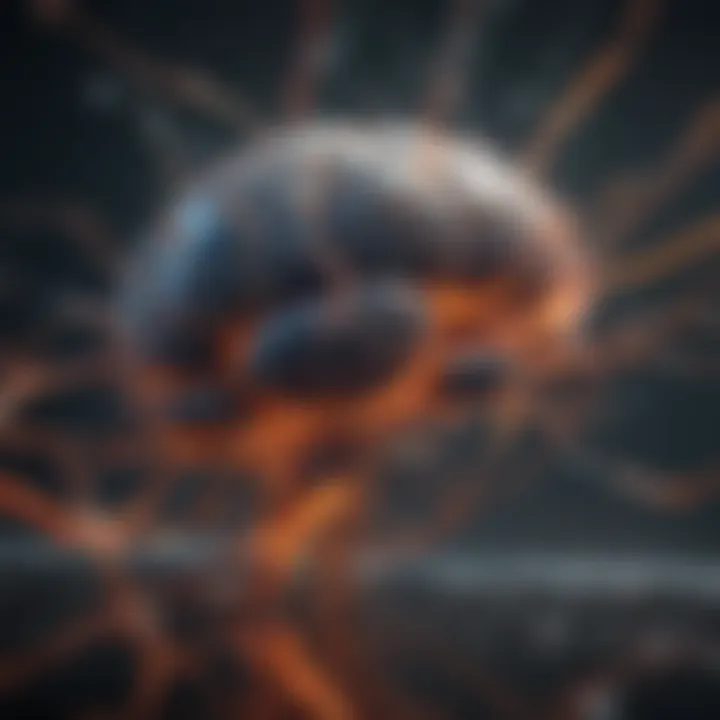
Supervised learning processes
Supervised learning processes entail training AI models using labeled data to predict or classify output values accurately. This mechanism contributes significantly to enhancing AI performance by providing clear guidelines for learning and decision-making. The key characteristic of supervised learning lies in its ability to leverage existing knowledge to improve model accuracy and efficiency. This approach is popular in various AI applications for its structured learning framework and applicability in diverse domains. The unique feature of supervised learning processes is their comprehensiveness in learning complex patterns and relationships, offering valuable insights for data analysis and inference. Despite its advantages in optimizing model training, supervised learning processes may face limitations in processing unstructured data or adapting to evolving environments.
Unsupervised learning approaches
Unsupervised learning approaches involve training AI models on unlabeled data to identify patterns or relationships independently. This mechanism contributes to exploring hidden structures within data and discovering meaningful insights without explicit guidance. The key characteristic of unsupervised learning lies in its capacity to uncover novel information and trends through autonomous learning processes. This approach is favored for its versatility in exploratory data analysis and anomaly detection across various datasets. The unique feature of unsupervised learning approaches is their adaptability to diverse data types and complexity levels, offering flexibility in pattern recognition and data clustering. While beneficial for uncovering underlying data distributions, unsupervised learning may face challenges in interpreting results accurately and generalizing learning outcomes effectively.
Plasticity in Synaptic AI
Synaptic plasticity concepts
Synaptic plasticity concepts refer to the ability of neural connections to adapt and modify their strength based on experiences and stimuli. This concept underpins the learning and memory capabilities of AI systems, enabling them to adjust synaptic connections dynamically. The key characteristic of synaptic plasticity concepts is their role in promoting learning efficiency and cognitive flexibility in AI models. This aspect is beneficial for refining neural network functionalities and optimizing performance in dynamic environments. The unique feature of synaptic plasticity concepts is their capacity for continuous improvement and adaptability, fostering resilience and robustness in AI learning processes. However, the complexity of synaptic plasticity mechanisms may require sophisticated algorithms and training strategies to achieve optimal learning outcomes.
Long-term potentiation (LTP)
Long-term potentiation (LTP) signifies the persistent strengthening of synaptic connections resulting from repeated neural stimulation. This phenomenon plays a vital role in encoding memory and behavior in AI systems, enhancing their learning and decision-making capabilities. The key characteristic of LTP lies in its correlation with sustained synaptic enhancements and information retention in neural networks. This feature is instrumental in promoting long-term learning and knowledge retention across AI models. The unique feature of LTP is its impact on forming stable neural pathways and facilitating efficient information processing in AI algorithms. While advantageous for long-term learning processes, LTP mechanisms may encounter challenges in managing synaptic overexcitation and balancing neural activity effectively.
Advanced Applications:
Cognitive Computing:
Problem-solving capabilities:
The Problem-solving capabilities of Synaptic AI within the realm of Cognitive Computing play a pivotal role in streamlining decision-making processes and enhancing operational efficiency. By harnessing the power of advanced algorithms and neural networks, Cognitive Computing empowers machines to simulate human-like problem-solving skills, offering a unique advantage in addressing intricate challenges with speed and accuracy. The key characteristic of Problem-solving capabilities lies in their ability to adapt and learn from data, enabling continuous optimization and innovation. This feature proves beneficial for this article as it underscores the transformational potential of Cognitive Computing in revolutionizing traditional problem-solving approaches.
Natural language processing:
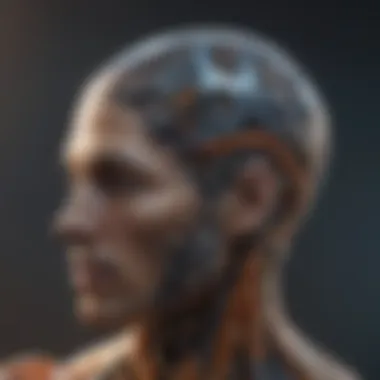
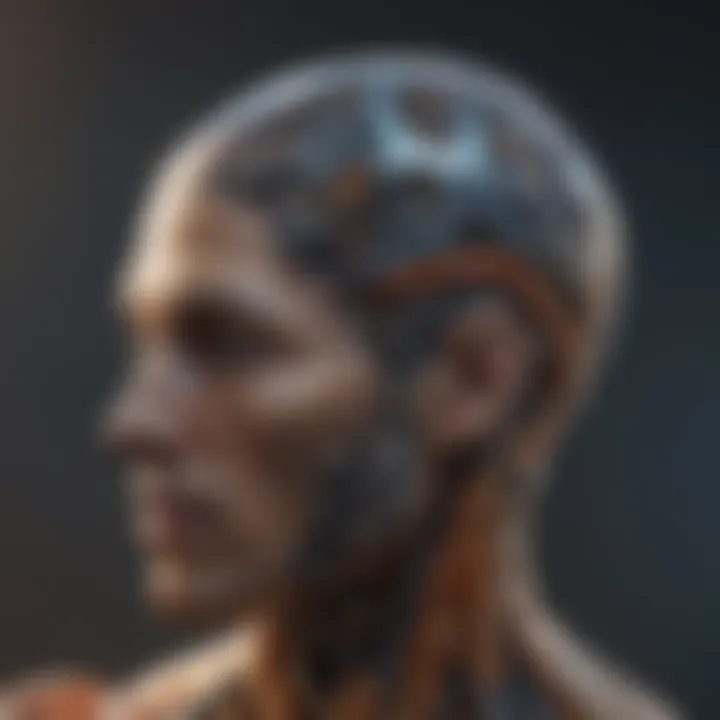
Natural Language Processing (NLP) within Cognitive Computing extends the capabilities of Synaptic AI by enabling machines to comprehend, interpret, and generate human language effortlessly. The integration of NLP into Synaptic AI enhances user engagement, facilitates data analysis, and streamlines information retrieval processes. The unique feature of NLP lies in its ability to decipher context, sentiment, and intent from human language inputs, leading to more personalized and intuitive interactions. While NLP offers significant advantages in enhancing communication and automation, its complexity and reliance on vast datasets pose challenges in ensuring accuracy and scalability within Cognitive Computing applications.
Neuroscience Insights:
Understanding brain functionality:
The Understanding brain functionality aspect of Synaptic AI delves into the intricate mechanisms of the human brain to replicate and optimize cognitive processes in artificial intelligence systems. By elucidating how the brain processes information, adapts to stimuli, and forms connections, Synaptic AI gains valuable insights for enhancing learning algorithms, decision-making processes, and pattern recognition abilities. The key characteristic of Understanding brain functionality is its emphasis on mimicking neurobiological processes to achieve higher levels of intelligence and efficiency in AI applications. This feature proves beneficial for this article as it underscores the transformative potential of bridging neuroscience with artificial intelligence to unlock new frontiers in cognitive computing.
Mapping neural connections:
Mapping neural connections involves the meticulous process of tracing and analyzing the intricate network of synapses within biological brains to inform the design and optimization of neural networks in AI systems. This insight plays a crucial role in enhancing the performance, scalability, and adaptability of Synaptic AI by mimicking the interconnected structure of the brain. The key characteristic of Mapping neural connections lies in its ability to enhance the efficiency and accuracy of information processing within AI architectures, leading to enhanced learning capabilities and cognitive functionalities. While this approach offers advantages in optimizing AI performance and driving innovation, the complexity of neural mapping and the computational resources required pose challenges in implementation and scalability within AI systems.
Challenges and Future Prospects
In the realm of synaptic AI, addressing challenges and exploring future prospects is paramount for advancing the field. By delving into the obstacles that lie ahead and envisioning the potential trajectories, practitioners can steer the course of synaptic AI development towards greater efficacy and ethical considerations. The nexus of challenges and future prospects not only shapes the evolution of AI technologies but also influences societal norms and ethical frameworks. Understanding and proactively engaging with these aspects are essential for harnessing the full power of synaptic AI.
Ethical Considerations
Privacy concerns in AI
Within the domain of AI, privacy concerns stand out as a critical facet that demands attention and meticulous management. Privacy concerns in AI pertain to the safeguarding of sensitive data, ensuring data protection, and preventing unauthorized access or misuse. This specific aspect underscores the need for robust data security measures and transparent data handling practices to uphold individual and collective privacy rights. By addressing privacy concerns in AI, organizations can foster trust among users and establish ethical standards that align with regulatory requirements and societal expectations, thereby enhancing the credibility and sustainability of AI applications.
Ethical algorithm development
Ethical algorithm development signifies a conscientious approach to designing and implementing algorithms that prioritize ethical principles and moral values. This aspect emphasizes the significance of integrating ethical considerations into the algorithmic decision-making process, ensuring fairness, accountability, and transparency. Ethical algorithm development contributes to mitigating biases, minimizing harm, and promoting equity in AI systems. By embedding ethical principles into algorithm development, stakeholders can foster responsible AI innovations that resonate with ethical frameworks and societal values, ultimately enhancing the ethical landscape of AI and fostering sustainable technology adoption.
Advancements on the Horizon
Enhanced cognitive computing
The advancement of cognitive computing heralds a new era of AI capabilities, accentuating cognitive functionalities that mimic human thought processes and reasoning. Enhanced cognitive computing entails the augmentation of AI systems with advanced reasoning, inference, and problem-solving abilities, enabling machines to comprehend complex information, learn iteratively, and adapt dynamically. This specific aspect propels AI towards cognitive autonomy, enabling machines to simulate human-like cognitive functions such as decision-making, pattern recognition, and natural language understanding. Enhanced cognitive computing holds the promise of revolutionizing industries, enhancing user experiences, and driving innovation in cognitive technologies, thereby reshaping the future landscape of AI applications.
Integration with robotics
The integration of AI with robotics represents a symbiotic relationship that amalgamates cognitive computing with physical automation, creating intelligent robotic systems with adaptive learning capabilities. Integration with robotics empowers machines to perceive, interact, and collaborate in real-world environments, transcending traditional automation limits. This specific aspect underscores the convergence of AI and robotics to enhance operational efficiency, streamline processes, and augment human-machine interactions. By integrating AI with robotics, organizations can leverage smart robotics solutions that optimize productivity, enhance safety, and drive technological advancements, ushering in a new era of human-robot collaboration and innovative applications.